Mideast & Africa News
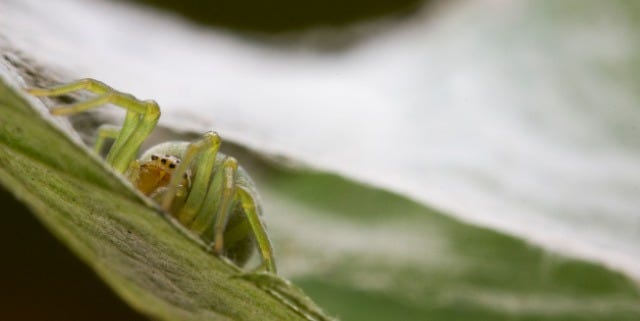
Critical GitLab Bug Under Exploit Enables Account Takeover, CISA Warns
Critical GitLab Bug Under Exploit Enables Account Takeover, CISA Warns
Patch now: Cyberattackers are exploiting CVE-2023-7028 (CVSS 10) to take over and lock users out of GitLab accounts, steal source code, and more.
Latest Commentary
Global Director, Cybersecurity Architecture and Engineering, Estee Lauder Companies
Co-Founder & CEO, Eureka Security
Deep Reading
See More Dark Reading ResearchFeeling creative? Submit your caption and our panel of experts will reward the winner with a $25 Amazon gift card.
As the social media giant celebrates its two-decade anniversary, privacy experts reflect on how it changed the way the world shares information.
The semiconductor manufacturing giant's security team describes how hardware hackathons, such as Hack@DAC, have helped chip security by finding and sharing hardware vulnerabilities.
Cybersecurity Features In-Depth: On security strategy, latest trends, and people to know. Brought to you by Mandiant.
Security Technology: Featuring news, news analysis, and commentary on the latest technology trends.
The new company will focus on cybersecurity services as a top 10 managed security service provider, but must expand outside the low-margin management of security into detection and response.
Microsoft is previewing new AI and machine learning capabilities in Defender XDR that will help detect and block malicious OAuth applications.
The startup's new identity and access management platform uncovers poorly monitored service accounts and secures them from abuse.
The growing amount of surveillance technology being deployed in the country is concerning due to Indonesia's increasing blows to citizens' civil rights.
A recent campaign targeting Middle Eastern government organizations plays standard detection tools like a fiddle. With cyberattackers getting more creative, defenders must start keeping pace.
Some customers found that they had the ability to cancel a stranger's flight to another country after opening the app, which was showing other individuals' flight details.
Breaking cybersecurity news, news analysis, commentary, and other content from around the world.
Partner Perspectives
More Partner PerspectivesPress Releases
See allBlack Hat USA - August 3-8 - Learn More
August 3, 2024Cybersecurity's Hottest New Technologies: What You Need To Know
March 21, 2024
Key Findings from the State of AppSec Report 2024
May 7, 2024Is AI Identifying Threats to Your Network?
May 14, 2024Where and Why Threat Intelligence Makes Sense for Your Enterprise Security Strategy
May 15, 2024Safeguarding Political Campaigns: Defending Against Mass Phishing Attacks
May 16, 2024Why Effective Asset Management is Critical to Enterprise Cybersecurity
May 21, 2024