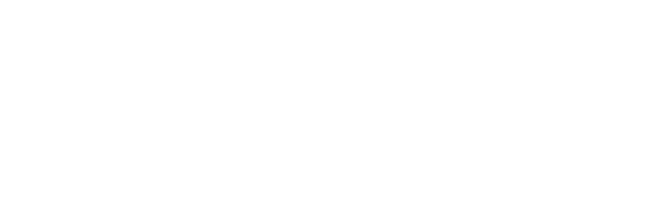
News, news analysis, and commentary on the latest trends in cybersecurity technology.
Improve Confidence and Context to Sell the SOC on Automating
SIEM and XDR made a lot of promises, but they don't quite live up to the SOC team's standards.
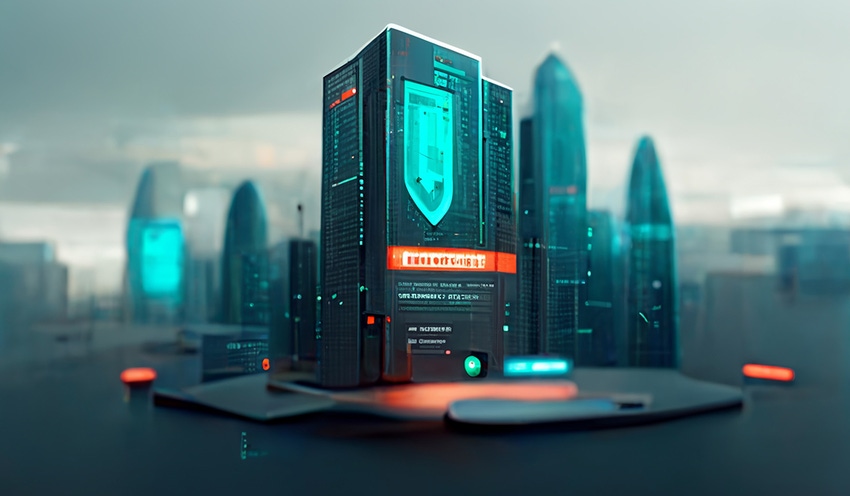
One might think that security teams are automating various phases of the SOC lifecycle, looking forward to saving time and speeding up mean time to detection (MTTD) and mean time to response (MTTR). But in reality, security teams don't have confidence in automation because of too many false positives, poor detection, a lack of complete analytics, and the fact that the analytics available to them are siloed between different detection tools and not linked together effectively.
These factors lead to poor-quality response playbooks from threat detection, investigation, and response (TDIR) solutions that can't be trusted. Without confidence that the response will eliminate the threat without disrupting other important business processes, security teams won't be comfortable with automation in the SOC.
Most TDIR solutions fall short of this confidence threshold because they don't gather enough contextual information around a threat and thus don't customize their response playbook to the situation and their environment. The security analyst will need to manually gather contextual information and decide how to tailor the playbook before passing it off to the appropriate team to implement. That all takes time, which further reduces MTTD and MTTR.
So, what is missing from TDIR solutions that causes this lack of confidence? Let's explore four ways that current SIEM and XDR solutions fail to live up to the SOC team's standards for confidence and context, and some ways they could do better.
Problem: Can't Automatically Scale to Accept a High Volume of Data
Being able to ingest as much data from as many sources as possible means the system can provide more context along with alerts and make remediations more targeted. Without this extra data, the response usually is not specific enough for the SOC team to be comfortable proceeding without re-checking everything manually.
Unfortunately, many SIEMs charge based on the volume of data the solution takes in, which creates a trade-off between cost and data. In this situation, getting enough data to help the analyst might be too expensive!
Solution: Choose a solution with a different pricing plan, like charging per user and/or per device rather than by data volume. This ensures the cost will stay relatively consistent even if data volumes change significantly (which they often do).
Problem: Can't Automatically Ingest and Interpret Data from Many Different Sources
Now that we've established that a high intake of data is essential for increasing the SOC team's confidence in responses, being able to process and sort that data is the second half of the equation. The SIEM must be able to process both structured and unstructured data with some type of data interpretation engine. The more integrations the SIEM has out of the box, the better.
Solution: The ability to ingest unstructured data, parse it, and extract useful information from it significantly increases a SIEM's threat detection capabilities. For example, data from HR systems can help identify insider threats and potentially disgruntled employees, but this is generally not structured in a format a SIEM can process.
Problem: Can't Execute True Machine Learning and Advanced Security Analytics
True machine learning (ML) capabilities — as in trained ML rather than rule-based ML — make threat detection more accurate, which in turn makes response playbooks more targeted and customized. Threat detection based on trained ML can better detect new and unknown attacks and adjust to changing network behavior without needing to be updated. Rule-based ML is like a flowchart; its inputs and outputs are fixed, and therefore it is limited in its findings and relatively poor in accuracy. When a new, never-before-seen cyberattack appears in the wild, a rule-based detection solution won't be able to detect it until the definitions get updated, which can take days or even weeks depending on how responsive the vendor is.
Solution: An ML program should recognize and flag the attack as a potential threat based on contextual data. Better accuracy means the SOC team will be more confident, which means they'll need to do less manual investigating.
Problem: Can't Automatically Validate Findings and Create Detailed Risk Scores
The ability to prioritize responses based on risk is the final piece of the puzzle for improving the SOC team's confidence. Many SIEM or XDR products give a generic risk score based on CVE and CVSS scores (if they provide one at all). These scores generally aren't customized to their environment.
Solution: More advanced solutions will generate a risk score based on data from vulnerability scanning tools, user access info, HR applications, etc. For example, take a user talking to an unknown external site for the first time. How risky is this? If that external site is known to host malware (identified via reputational services) or that user that was recently put on a performance plan and might have a grudge against the company, the risk is high. On the other hand, a user logging into company resources from an unknown IP address is less risky if that user is working remotely.
Assessing risk in this way is difficult and requires a lot of contextual data, but it allows the SIEM to automatically identify high-risk attacks and helps the SOC team to trust that decision, which means a more streamlined and effective process.
Improve the Process
Fast responses are desired in cases with a high-risk security event and a low-impact response. Automating parts of the SOC process and getting better quality data from the SIEM helps the security team assess the context and risk score quickly and confidently. In turn, this means faster responses and a safer organization. But until TDIR solutions can improve in these four areas, security teams will continue to lack confidence in automation in the SOC.
About the Author(s)
You May Also Like
Key Findings from the State of AppSec Report 2024
May 7, 2024Is AI Identifying Threats to Your Network?
May 14, 2024Where and Why Threat Intelligence Makes Sense for Your Enterprise Security Strategy
May 15, 2024Safeguarding Political Campaigns: Defending Against Mass Phishing Attacks
May 16, 2024Why Effective Asset Management is Critical to Enterprise Cybersecurity
May 21, 2024
Black Hat USA - August 3-8 - Learn More
August 3, 2024Cybersecurity's Hottest New Technologies: What You Need To Know
March 21, 2024