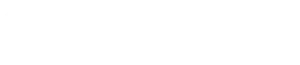
Enterprise cybersecurity technology research that connects the dots.
Understanding the Omdia Threat Detection Data Life Cycle
Data quality is key in an effective TDIR solution. Omdia's threat detection data life cycle highlights the considerations for effective data-driven threat detection.
July 12, 2022
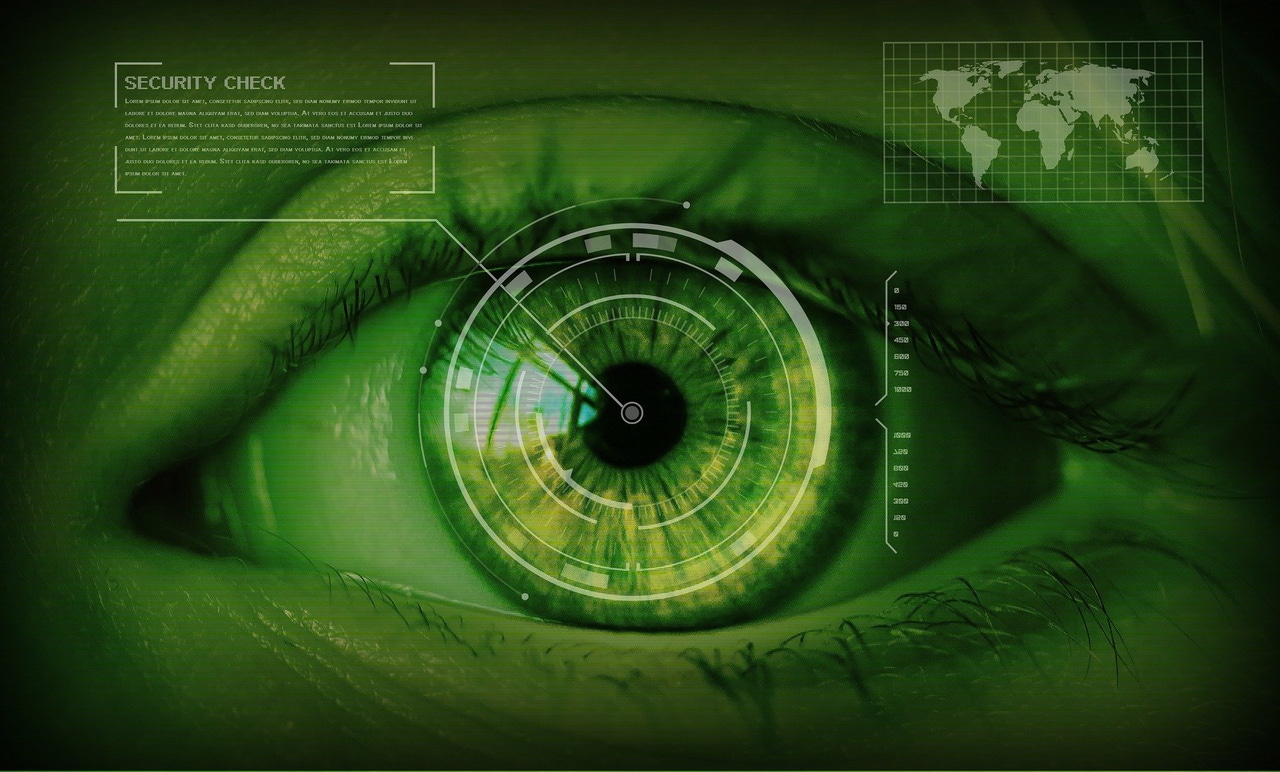
Threat detection, investigation, and response (TDIR) solutions all rely on data to deliver accurate, consistent, and performant threat detection, prioritization, and analysis. Enterprises need good data from the right places, refined and applied in the right ways, to detect and ultimately mitigate threats.
For that reason, Omdia believes taking a data-driven life-cycle approach is the best strategy to ensure data-related elements of the TDIR process are effective.
Below is a brief review of the steps in the Omdia threat detection data life cycle, a multistage process through which enterprise cybersecurity operations (SecOps) leaders may consider the tactical implications of how data is utilized by their TDIR solutions for the purpose of threat detection.
Acquisition: Identify the relevant data types or sources pertinent to the threat detection process, confirm the location of the data, and identify the steps for acquiring this data, both technical and business.
Ingestion: Threat detection data must be confirmed as valid and permitted for the system where it will be utilized, and then ingested in streaming real-time mode or batched and ingested at intervals based on different factors.
Processing: Unprocessed logs are analyzed in detail to determine key characteristics, such as origin, source format or schema, and data values or elements. It is often necessary to reformat or parse the logs into a preferred format to ensure consistency and accelerate other steps in the life cycle. After parsing, data is validated to ensure it conforms to system parameters.
Normalization: Unnecessary, and redundant data is deduplicated, reduced, and/or removed; new solution-specific fields are added, and the output is further standardized with common metadata classifiers. Logs that enter the system with significant variances are adjusted to appear similar.
Bypassing normalization: Some threat detection data systems intentionally do not conduct a normalization stage. In this scenario, the normalization step is skipped, and data moves directly from processing into categorization.
Categorization: The contents of the data are further examined to identify which established system attributes should be assigned to the data. The purpose of categorization is to delineate the contextual relevance of the data during subsequent analysis.
Enrichment: New data is augmented with additional data attributes that add context or create logical connections to other data, system-defined attributes, or events. In nearly all instances effective enrichment is driven at least in part by analytics, technology that analyzes data over time, identifies patterns in the data, and creates a baseline of so-called "normal" or expected activity for a given use case.
Indexing: Data is added to an index that denotes where it is located within the storage system. An index exists to optimize the performance of the system when the data is accessed.
Storage: Data then enters the storage phase, typically for a definite period, based on policy. Contemporary TDIR solutions increasingly rely on cloud-based data-lake technology, residing either directly in a public cloud environment or in a third-party environment managed by the vendor or provider.
Analysis: Once added to the dataset, data is analyzed on an ongoing basis. Many TDIR solutions reanalyze the existing dataset when new data is added. Analysis also occurs on a per query basis, as well as for proactive threat hunting.
Valuation: Process by which the business value of all lifecycle data is evaluated on an ongoing basis in support of TDIR process improvement or desired business outcomes.
Omdia believes achieving different, better results from TDIR requires the implementation of different, better approaches within the threat detection data life cycle.
Though there are inherent challenges with the life cycle, especially in the areas of data processing and normalization, there are also fascinating innovations taking root, particularly customized categorization schemas (nonstandard indexing to accelerated data analysis) and security data lake-houses (storage environments that combine the best of data lakes and data warehouse).
Regardless, a process-centric approach to the threat detection data life cycle with careful attention to detail will provide better, more consistent TDIR results, and set the stage for further data life-cycle innovation.
About the Author(s)
You May Also Like
CISO Perspectives: How to make AI an Accelerator, Not a Blocker
August 20, 2024Securing Your Cloud Assets
August 27, 2024