Unpatched Critical Vulnerabilities Open AI Models to Takeover
The security holes can allow server takeover, information theft, model poisoning, and more.
November 15, 2023
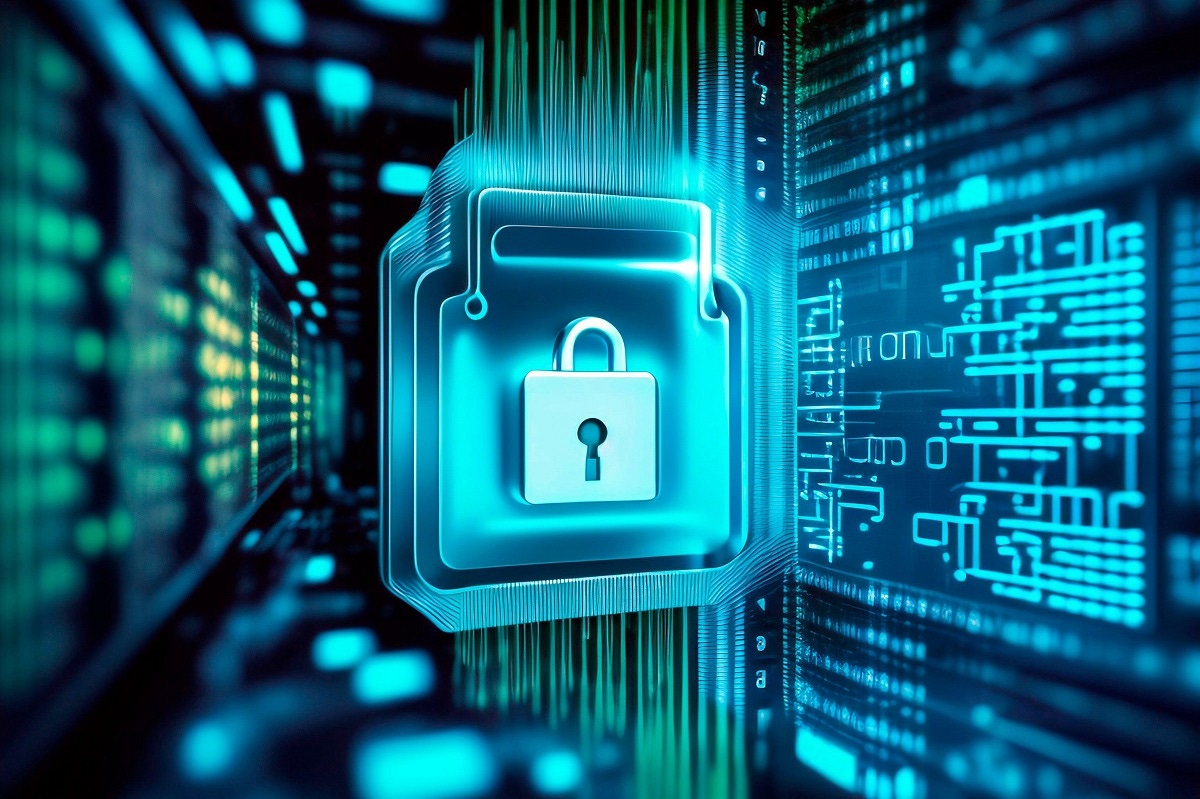
Researchers have identified nearly a dozen critical vulnerabilities in the infrastructure used by AI models (plus three high- and two medium-severity bugs), which could leave companies at risk as they race to take advantage of AI. Some of them remain unpatched.
The affected platforms are used for hosting, deploying, and sharing large language models (LLM), and other ML platforms and AIs. They include Ray, used in the distributed training of machine-learning models; MLflow, a machine-learning lifecycle platform; ModelDB, a machine-learning management platform; and H20 version 3, an open source platform for machine learning based on Java.
Machine-learning security firm Protect AI disclosed the results on Nov. 16 as part of its AI-specific bug-bounty program, Huntr. It notified the software maintainers and vendors about the vulnerabilities, allowing them 45 days to patch the issues.
Each of the issues has been assigned a CVE identifier, and while many of the issues have been fixed, others remain unpatched, in which case Protect AI recommended a workaround in its advisory.
AI Bugs Present High Risk to Organizations
According to Protect AI, vulnerabilities in AI systems can give attackers unauthorized access to the AI models, allowing them to co-opt the models for their own goals.
But, they can also give them a doorway into the rest of the network, says Sean Morgan, chief architect at Protect AI. Server compromise and theft of credentials from low-code AI services are two possibilities for initial access, for example.
"Inference servers can have accessible endpoints for users to be able to use ML models [remotely], but there are a lot of ways to get into someone's network,” he says. "These ML systems that we're targeting [with the bug-bounty program] often have elevated privileges, and so it's very important that if somebody's able to get into your network, that they can't quickly privilege escalate into a very sensitive system."
For instance, a critical local file-inclusion issue (now patched) in the API for the Ray distributed learning platform allows an attacker to read any file on the system. Another issue in the H20 platform (also fixed) allows code to be executed via the import of a AI model.
The risk is not theoretical: Large companies have already embarked on aggressive campaigns to find useful AI models and apply them to their markets and operations. Banks already use machine learning and AI for mortgage processing and anti-money laundering, for example.
While finding vulnerabilities in these AI systems can lead to compromise of the infrastructure, stealing the intellectual property is a big goal as well, says Daryan Dehghanpisheh, president and co-founder of Protect AI.
"Industrial espionage is a big component, and in the battle for AI and ML, models are a very valuable intellectual property asset," he says. "Think about how much money is spent on training a model on the daily basis, and when you're talking about a billion parameters, and more, so a lot of investment, just pure capital that is easily compromised or stolen."
Battling novel exploits against the infrastructure underpinning natural-language interactions that people have with AI systems like ChatGPT will be even more impacting, says Dane Sherrets, senior solutions architect at HackerOne. That's because when cybercriminals are able to trigger these sorts of vulnerabilities, the efficiencies of AI systems will make the impact that much greater.
These attacks "can cause the system to spit out sensitive or confidential data, or help the malicious actor gain access to the backend of the system," he says. "AI vulnerabilities like training data poisoning can also have a significant ripple effect, leading to widespread dissemination of erroneous or malicious outputs."
Security for AI Infrastructure: Often Overlooked
Following the introduction of ChatGPT a year ago, technologies and services based on AI — especially generative AI (GenAI) — have taken off. In its wake, a variety of adversarial attacks have been developed that can target AI and machine-learning systems and their operations. On Nov. 15, for example, AI security firm Adversa AIdisclosed a number of attacks on GPT-based systems including prompt leaking and enumerating the APIs to which the system has access.
Yet, ProtectAI's bug disclosures underscore the fact that the tools and infrastructure that support machine-learning processes and AI operations can also become targets. And often, businesses have adopted AI-based tools and workflows without often consulting information security groups.
"As with any high-tech hype cycle, people will deploy systems, they'll put out applications, and they'll create new experiences to meet the needs of the business and the market, and often will either neglect security and they create these kinds of 'shadow stacks,' or they will assume that the existing security capabilities they have can keep them safe," says Dehghanpisheh. "But the things we [cybersecurity professionals] are doing for traditional data centers, don't necessarily keep you safe in the cloud, and vice versa."
Protect AI used its bug bounty platform, dubbed Huntr, to solicit vulnerability submissions from thousands of researchers for different machine-learning platforms, but so far, bug hunting in this sector remains in its infancy. That could be about to change, though.
For instance, Trend Micro's Zero Day Initiative has not seen significant demand yet for finding bugs in AI/ML tools, but the group has seen regular shifts in what types of vulnerabilities the industry wants researchers to find, and an AI focus will likely be coming soon, says Dustin Childs, Head of Threat Awareness at Trend Micro's Zero Day Initiative.
"We're seeing the same thing in AI that we saw in other industries as they developed," he says. "At first, security was de-prioritized in favor of adding functionality. Now that it's hit a certain level of acceptance, people are starting to ask about the security implications."
About the Author
You May Also Like