Redefining Cyber Defense: Fighting Emerging Threats With AI
To combat the ever-evolving threat landscape, defenders need artificial intelligence (AI)-native technology.
January 29, 2024
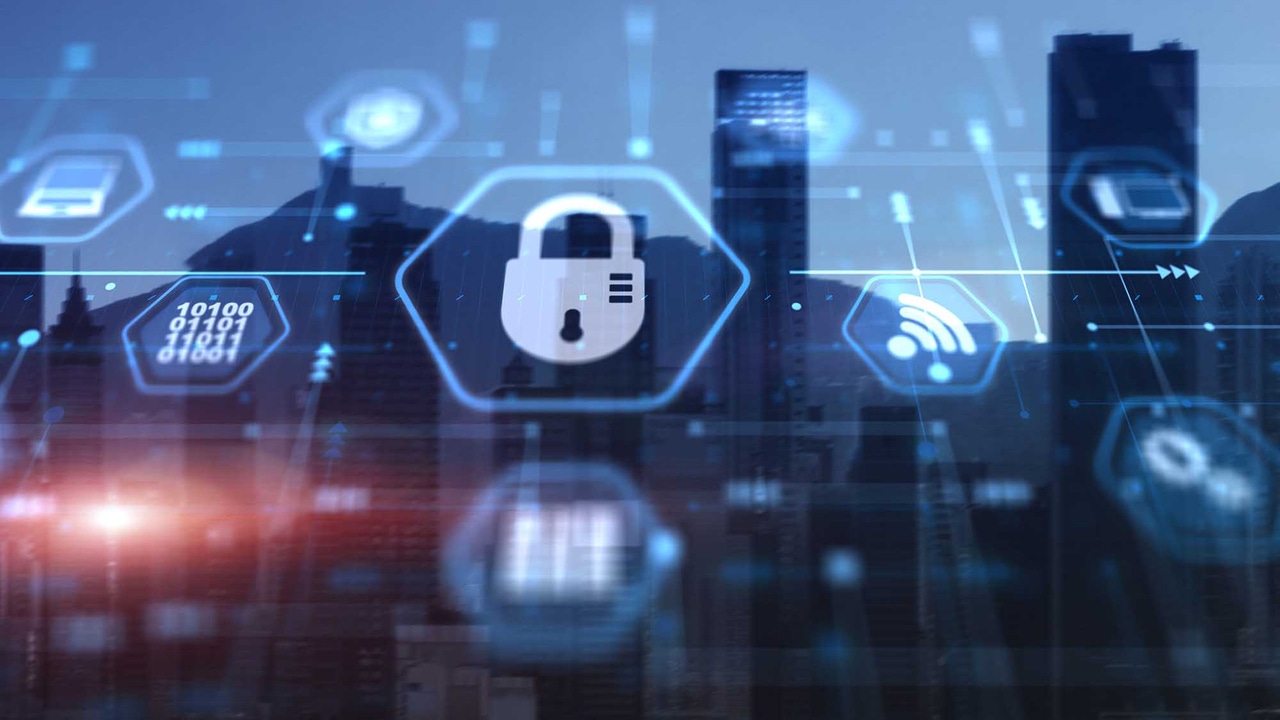
By Jack Stockdale, Founding CTO, Darktrace
Over the past year, the integration of artificial intelligence (AI) into daily life, fueled by tools like ChatGPT, has surged. In cybersecurity, adversarial adoption of AI presents challenges.
There are three potential areas for AI to boost attackers: increasing the sophistication of low-level threat actors, increasing the speed of attacks through automation, and eroding trust among users.
AI Changes the Cyber-Threat Landscape
We've already seen potential indicators of these shifts.
In April 2023, Darktrace reported a 135% rise in "novel social engineering attacks" coinciding with ChatGPT's popularity surge, indicating AI tools may enable more sophisticated phishing attacks at a rapid pace.
From May to July, Darktrace found a 59% uptick in multistage payload attacks, with July seeing 50,000 more such attacks than May, indicating potential use of automation. The speed of these types of attacks will likely continue to rise as attackers adopt more AI tools.
In the same period, Darktrace observed changes in attacks that abuse trust. While email impersonation of executives dropped by 11%, email account takeovers rose by 52%, and IT team impersonations rose by 19%. These changes suggest that employees are becoming used to VIP impersonation, so attackers are pivoting to IT team impersonation. Generative AI has the potential to increase linguistic sophistication and can also be used to produce realistic voice deepfakes to aid attackers in their deceptions.
These statistics suggest a new era of disruption and obstacles for cybersecurity — an era in which novel is the new normal.
AI's Evolving Role in Cybersecurity
Most cybersecurity AI today is trained periodically in offline training environments on huge amounts of combined historic data. After a few days or weeks, you get a static AI model that you push live to serve its role until the next version is ready. This type of AI doesn't learn and updates only once the next version is pushed live.
This application of AI cannot protect an organization from the AI-powered cyber-threat landscape. The rate and sophistication of new threats outpace a history-based defense, leaving organizations vulnerable to the AI-powered threats we're already seeing today, as well as the threats of tomorrow.
Yet alternative approaches of AI in cybersecurity can help keep up with evolving threats. It comes down to the type of AI, the data it is trained on, and most importantly, how the two interact.
Instead of bringing their data to the AI, teams should bring AI to their data. This means every organization will have a unique AI engine that is plugged into their enterprise to train and self-learn on their data in real time across cloud environments, email systems, networks, operational systems, and physical locations.
This organization-centric approach, with tailored models and training data, can mean the difference between stopping or succumbing to novel attacks. By understanding what is normal for a specific organization across its devices and users, the AI can recognize abnormal activity that indicates a cyberattack and stop it.
This approach to AI has worked in the past, as self-learning AI successfully detected and protected against novel attacks including:
Supply chain attacks like SolarWinds
Zero-day exploits like Citrix Netscaler
Novel ransomware worms like WannaCry
Sophisticated nation-state attacks like APT35
This application of AI protected businesses not because it was looking specifically for these threats, but because every threat, whether known or novel, accidental or malicious, human or AI-driven, impacts the organization, its people, and its data.
Applying the Right AI for the Right Job
AI-based cybersecurity that can detect and stop novel and AI-powered attacks is not achieved with just one type of AI, but a robust combination. These types include:
A wide range of self-learning methods to understand new information and decide if something never seen before looks suspicious.
Real time Bayesian probabilistic methods, which allow models to be efficiently updated and controlled in real time.
Deep-neural networks to replicate the thought process of humans.
Graph theory, which understands the incredibly complex relationships between people, systems, organizations, and supply chains.
Offensive AI techniques such as generative adversarial networks (GANs) to help test and improve the ability to counter AI-driven attacks.
Natural language processing and large language models to interpret and produce human-consumable output.
Just as AI brings new challenges to the cyber-threat landscape, it also facilitates new ways to counter them. By adopting AI techniques trained on the organization's unique data, security teams can boost defenses with protection that is always on, always learning, and always ready to stop any attacks.
About the Author:
Jack Stockdale is the founding CTO at Darktrace. With over 20 years' experience in software engineering, Jack is responsible for overseeing the development of Bayesian mathematical models and artificial intelligence algorithms that underpin Darktrace's award-winning technology. Jack and his development team in Cambridge were recognized for their outstanding contribution to engineering by the Royal Academy of Engineering MacRobert Innovation Award Committee in 2017 and again in 2019. Jack has a degree in computer science from Lancaster University.
Read more about:
Sponsor Resource CenterYou May Also Like
CISO Perspectives: How to make AI an Accelerator, Not a Blocker
August 20, 2024Securing Your Cloud Assets
August 27, 2024