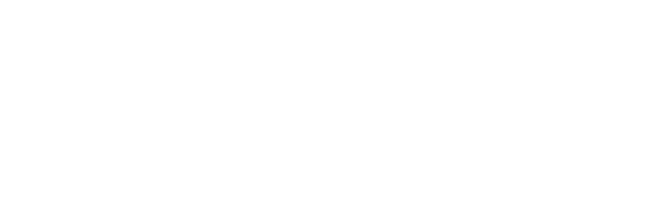
Cybersecurity In-Depth: Feature articles on security strategy, latest trends, and people to know.
How to Safely Architect AI in Your Cybersecurity Programs
Guardrails need to be set in place to ensure confidentiality of sensitive information, while still leveraging AI as a force multiplier for productivity.
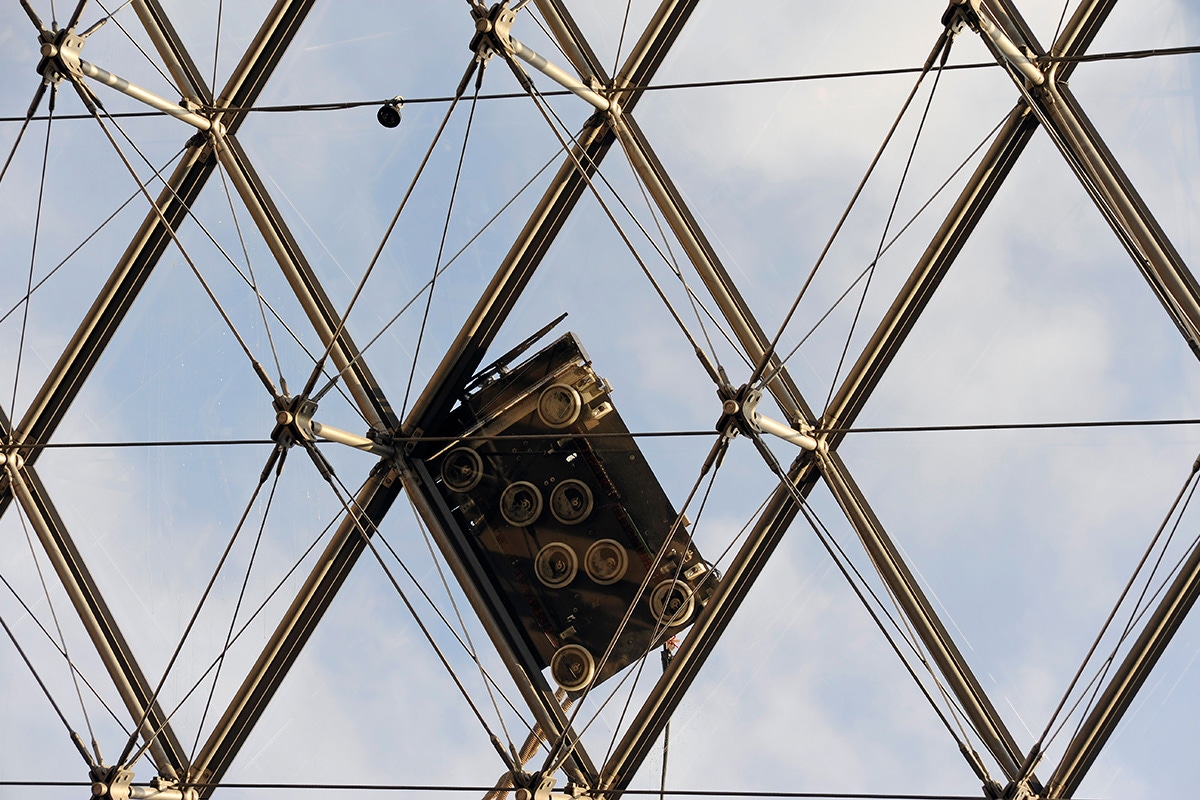
At the end of June, cybersecurity firm Group-IB revealed a notable security breach that impacted ChatGPT accounts. The company identified a staggering 100,000 compromised devices, each with ChatGPT credentials that were subsequently traded on illicit Dark Web marketplaces over the course of the past year. This breach prompted calls for immediate attention to address the compromised security of ChatGPT accounts, since search queries containing sensitive information become exposed to hackers.
In another incident, within a span of less than a month, Samsung suffered three documented instances in which employees inadvertently leaked sensitive information through ChatGPT. Because ChatGPT retains user input data to improve its own performance, these valuable trade secrets belonging to Samsung are now in the possession of OpenAI, the company behind the artificial intelligence (AI) service. This poses significant concerns regarding the confidentiality and security of Samsung's proprietary information.
Because of such worries about ChatGPT's compliance with the EU's General Data Protection Regulation (GDPR), which mandates strict guidelines for data collection and usage, Italy has imposed a nationwide ban on the use of ChatGPT.
Rapid advancements in AI and generative AI applications have opened up new opportunities for accelerating growth in business intelligence, products, and operations. But cybersecurity program owners need to ensure data privacy while waiting for laws to be developed.
Public Engine Versus Private Engine
To better comprehend the concepts, let's start by defining public AI and private AI. Public AI refers to publicly accessible AI software applications that have been trained on datasets, often sourced from users or customers. A prime example of public AI is ChatGPT, which leverages publicly available data from the Internet, including text articles, images, and videos.
Public AI can also encompass algorithms that use datasets not exclusive to a specific user or organization. Consequently, customers of public AI should be aware that their data might not remain entirely private.
Private AI, on the other hand, involves training algorithms on data that is unique to a particular user or organization. In this case, if you use machine learning systems to train a model using a specific dataset, such as invoices or tax forms, that model remains exclusive to your organization. Platform vendors do not use your data to train their own models, so private AI prevents any use of your data to aid your competitors.
Integrate AI Into Training Programs and Policies
In order to experiment, develop, and integrate AI applications into their products and services while adhering to best practices, cybersecurity staff should put the following policies into practice.
User Awareness and Education: Educate users about the risks associated with using AI and encourage them to be cautious when transmitting sensitive information. Promote secure communication practices and advise users to verify the authenticity of the AI system.
Data minimization: Provide the AI engine with only the minimum amount of data necessary to accomplish the task. Avoid sharing unnecessary or sensitive information that is not relevant to the AI processing.
Anonymization and de-identification: Whenever possible, anonymize or de-identify the data before inputting it into the AI engine. This involves removing personally identifiable information (PII) or any other sensitive attributes that are not required for the AI processing.
Secure Data Handling Practices: Establish strict policies and procedures for handling your sensitive data. Limit access to authorized personnel only and enforce strong authentication mechanisms to prevent unauthorized access. Train employees on data privacy best practices and implement logging and auditing mechanisms to track data access and usage.
Retention and Disposal: Define data retention policies and securely dispose of the data once it is no longer needed. Implement proper data disposal mechanisms, such as secure deletion or cryptographic erasure, to ensure that the data cannot be recovered after it is no longer required.
Legal and Compliance Considerations: Understand the legal ramifications of the data you are inputting into the AI engine. Ensure that the way users employ the AI complies with relevant regulations, such as data protection laws or industry-specific standards.
Vendor Assessment: If you are using an AI engine provided by a third-party vendor, perform a thorough assessment of the vendor's security measures. Ensure that it follows industry best practices for data security and privacy, and that it has appropriate safeguards in place to protect your data. ISO and SOC attestation, for example, provide valuable third-party validations of a vendor's adherence to recognized standards and their commitment to information security.
Formalize an AI Acceptable Use Policy (AUP): An AI acceptable use policy should outline the purpose and objectives of the policy, emphasizing the responsible and ethical use of AI technologies. It should define acceptable use cases, specifying the scope and boundaries for AI utilization. The AUP should encourage transparency, accountability, and responsible decision-making in AI usage, fostering a culture of ethical AI practices within the organization. Regular reviews and updates ensure the policy's relevance to evolving AI technologies and ethics.
Conclusions
By adhering to these guidelines, program owners can effectively leverage AI tools while safeguarding sensitive information and upholding ethical and professional standards. It is crucial to review AI-generated material for accuracy while simultaneously protecting the inputted data that goes into generating response prompts.
About the Author
You May Also Like
Unleashing AI to Assess Cyber Security Risk
Nov 12, 2024Securing Tomorrow, Today: How to Navigate Zero Trust
Nov 13, 2024The State of Attack Surface Management (ASM), Featuring Forrester
Nov 15, 2024Applying the Principle of Least Privilege to the Cloud
Nov 18, 2024The Right Way to Use Artificial Intelligence and Machine Learning in Incident Response
Nov 20, 2024