Vulnerability Remediation: Best Practice or Best Guess?
A new study from Kenna Security and the Cyentia Institute finds that even the most well-thought-out vulnerability remediation strategy is no better than a good guess. However, machine learning could lead to better results.
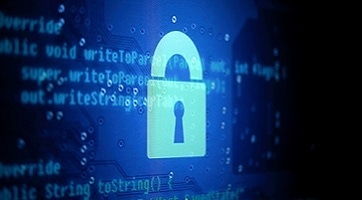
If you don't want to hear (structured) criticism of your vulnerability remediation strategy, close your ears now. Because chances are, enterprise security teams are doing no better statistically than random chance.
That's the startling finding of a new study from the Cyentia Institute and Kenna Security, a San Francisco-based predictive cyber risk firm. The two analyzed five years' worth of historical vulnerability data from 15 sources, and found that current remediation approaches to prioritizing and resolving vulnerabilities are about as effective -- even sometimes less effective -- than tackling issues in a random order.
It's not that remediation techs are doing a bad job once they've identified an issue, it's that deciding what order to tackle them is leaving enterprises open to damage from unpatched exploits further down the checklist.
(Source: Flickr)
"Effective remediation depends on quickly determining which vulnerabilities warrant action and which of those have highest priority, but prioritization remains one of the biggest challenges in vulnerability management," Kenna CEO Karim Toubba said.
"Businesses can no longer afford to react to cyber threats, as the research shows that most common remediation strategies are about as effective as rolling dice," Toubba added.
Predictive, not reactive
The concept of handling vulnerabilities remains unchanged: identify and remediate as rapidly as possible against an increasing number and velocity of threats. What's new is a change in posture that seeks to become predictive, rather than reactive. In past years, IT security has used analog tuning to try and identify and prioritize remediation, but this approach is now outmoded.
"Fast forward to 2018, and risk-based intelligent vulnerability management platforms now consume terabytes of configuration data, asset data, vulnerability data and threat intelligence to create a fine-grained analysis of which systems really need immediate patching against current threats," said Jon Oltsik, a senior principal analyst with the Enterprise Strategy Group.
Now there's a drive to move beyond real-time assessment of data into forecasting risks before an attack is possible. But of course, that's not easy.
Enterprises have an average of between 18 million and 24 million vulnerabilities across 60,000 assets, according to Cyentia. Every day of the year, they're faced with handling about 40 new vulnerabilities, and last year saw this number peak -- double that of 2016 -- and tracking to further grow this year.
The challenge is increased because most published vulnerabilities aren't used by attackers -- about 75% of known vulnerabilities never have an exploit developed for them, and then only 2% are ever used in an attack. As enterprises try to sort the wheat from the chaff, they're pressured because about half of new vulnerabilities are published within two weeks, effectively giving companies only ten working days to find them.
Now entering its fifth year, the 2020 Vision Executive Summit is an exclusive meeting of global CSP executives focused on navigating the disruptive forces at work in telecom today. Join us in Lisbon on December 4-6 to meet with fellow experts as we define the future of next-gen communications and how to make it profitable.
Essentially, this requires as wide a data input funnel as possible, filtered by a risk scoring model that provides results that increase the probability that a vulnerability will be exploited.
And the key to all of this seems to be machine learning.
Which vulnerabilities are hot?
"We use Machine Learning to comb through all the vulnerabilities previously released to figure out exactly what about a vulnerability makes it likely that an attacker would write an exploit for it," Michael Roytman, Kenna's chief data scientist, told SecurityNow. "We consider around 100,000 variables in doing so, and once we have a good idea of what those factors are, we make a best guess for every new vulnerability as it comes out."
He takes a leaf out of Charles Darwin's On the Origin of Species, constantly evolving the platform to adapt to new vulnerabilities which continuously pop up in the order of several million every 24 hours. Rather than using all of these inputs as training data for the platform -- meaning a risk the platform would never properly mature -- Roytman employs the concept of the survival of the fittest.
The performance of the current model is measured against a potential new one, using recent historical data. Whichever performs the best is taken forward for the next 24 hours. The "genetic origin" of today's model was created from selecting the best of 400 such initial models by giving each thousands of passes over an initial data set.
"We made every mistake imaginable, but as long as we understood how the algorithms worked, and as long as we kept a cool head and measured performance using sound statistical testing, we kept making steps in the right direction," he explained.
Related posts:
— Simon Marshall, Technology Journalist, special to Security Now
Read more about:
Security NowAbout the Author(s)
You May Also Like
Key Findings from the State of AppSec Report 2024
May 7, 2024Is AI Identifying Threats to Your Network?
May 14, 2024Where and Why Threat Intelligence Makes Sense for Your Enterprise Security Strategy
May 15, 2024Safeguarding Political Campaigns: Defending Against Mass Phishing Attacks
May 16, 2024Why Effective Asset Management is Critical to Enterprise Cybersecurity
May 21, 2024
Black Hat USA - August 3-8 - Learn More
August 3, 2024Cybersecurity's Hottest New Technologies: What You Need To Know
March 21, 2024