Looking Beyond the Hype Cycle of AI/ML in Cybersecurity
Artificial intelligence and machine learning aren't yet delivering on their cybersecurity promises. How can we close the gaps?
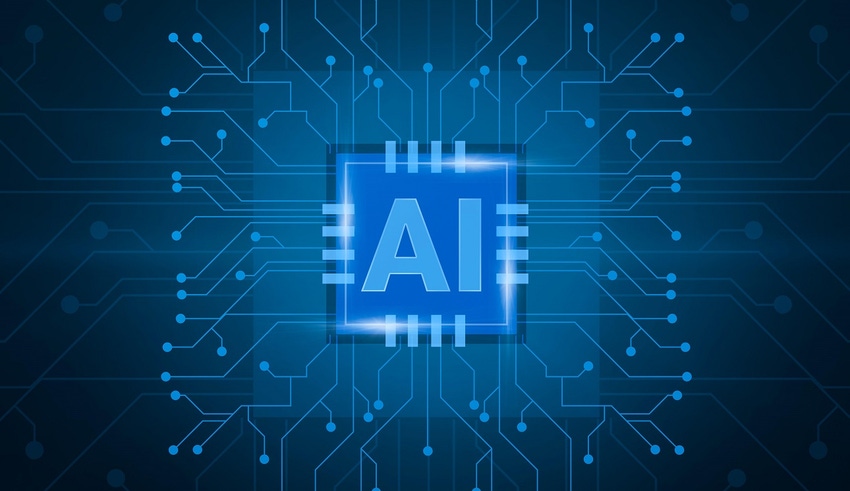
Most security teams can benefit from integrating artificial intelligence (AI) and machine learning (ML) into their daily workflow. These teams are often understaffed and overwhelmed by false positives and noisy alerts, which can drown out the signal of genuine threats.
The problem is that too many ML-based detections miss the mark in terms of quality. And perhaps more concerning, the incident responders tasked with responding to those alerts can't always interpret their meaning and significance correctly.
It's fair to ask why, despite all the breathless hype about the potential of AI/ML, are so many security users feeling underwhelmed? And what needs to happen in the next few years for AI/ML to fully deliver on its cybersecurity promises?
Disrupting the AI/ML Hype Cycle
AI and ML are often confused, but cybersecurity leaders and practitioners need to understand the difference. AI is a broader term that refers to machines mimicking human intelligence. ML is a subset of AI that uses algorithms to analyze data, learn from it, and make informed decisions without explicit programming.
When faced with bold promises from new technologies like AI/ML, it can be challenging to determine what is commercially viable, what is just hype, and when, if ever, these claims will deliver results. The Gartner Hype Cycle offers a visual representation of the maturity and adoption of technologies and applications. It helps reveal how innovative technologies can be relevant in solving real business problems and exploring new opportunities.
But there's a problem when people begin to talk about AI and ML. "AI suffers from an unrelenting, incurable case of vagueness — it is a catch-all term of art that does not consistently refer to any particular method or value proposition," writes UVA Professor Eric Siegel in the Harvard Business Review. "Calling ML tools 'AI' oversells what most ML business deployments actually do," Siegel says. "As a result, most ML projects fail to deliver value. In contrast, ML projects that keep their concrete operational objective front and center stand a good chance of achieving that objective."
While AI and ML have undoubtedly made significant strides in enhancing cybersecurity systems, they remain nascent technologies. When their capabilities are overhyped, users will eventually grow disillusioned and begin to question ML's value in cybersecurity altogether.
Another key issue hindering the broad deployment of AI/ML in cybersecurity is the lack of transparency between vendors and users. As these algorithms grow more complex, it becomes increasingly difficult for users to deconstruct how a particular decision was rendered. Because vendors often fail to provide clear explanations of their products' functionality citing confidentiality of their intellectual property, trust is eroded and users will likely just fall back on older, familiar technologies.
How to Fulfill the Cybersecurity Promise of AI and ML
Bridging the gulf between unrealistic user expectations and the promise of AI/ML will require cooperation between stakeholders with different incentives and motivations. Consider the following suggestions to help close this gap.
Bring security researchers and data scientists together early and often: Currently, data scientists may develop tools without fully grasping their utility for security, while security researchers might attempt to create similar tools but lack the necessary depth of knowledge in data science or ML. To unlock the full potential of their combined expertise, these two vastly different disciplines must work with and learn from each other productively. For instance, data scientists can enhance threat detection systems by using ML to identify meaningful patterns in large disparate datasets, while security researchers can contribute their understanding of threat vectors and potential vulnerabilities.
Use normalized data as the source: The quality of the data used to train models directly impacts the outcome and success of any AI/ML tool. In this increasingly data-driven world, the old adage "garbage in, garbage out" is truer than ever. As security shifts up to the cloud, normalizing telemetry at the point of collection means data is already in a standard format. Organizations can immediately stream normalized data into their detection cloud (a security data lake), making it easier to train and improve the accuracy of ML models without having to wrestle with format inconsistencies.
Prioritize the user experience: Security applications are not known for producing easy-to-use, streamlined user experiences. The only way to ship something people will use correctly is to start from the user experience rather than slapping it on at the end of the development cycle. By incorporating clean visualizations, customizable alert settings, and easy-to-understand notifications, security practitioners are more likely to adopt and engage with the tool. Likewise, it's essential to have a feedback loop when applying an AI/ML model to a security context so that security analysts and threat researchers can register their input and make corrections to tailor the model to their organization's requirements.
The ultimate goal of cybersecurity is to prevent attacks from happening rather than simply reacting to them after the fact. By delivering ML capabilities that security teams can put into practice, we can break the hype cycle and begin fulfilling its lofty promise.
About the Author(s)
You May Also Like
Beyond Spam Filters and Firewalls: Preventing Business Email Compromises in the Modern Enterprise
April 30, 2024Key Findings from the State of AppSec Report 2024
May 7, 2024Is AI Identifying Threats to Your Network?
May 14, 2024Where and Why Threat Intelligence Makes Sense for Your Enterprise Security Strategy
May 15, 2024Safeguarding Political Campaigns: Defending Against Mass Phishing Attacks
May 16, 2024
Black Hat USA - August 3-8 - Learn More
August 3, 2024Cybersecurity's Hottest New Technologies: What You Need To Know
March 21, 2024