Deception: Why It's Not Just Another Honeypot
The technology has made huge strides in evolving from limited, static capabilities to adaptive, machine learning deception.
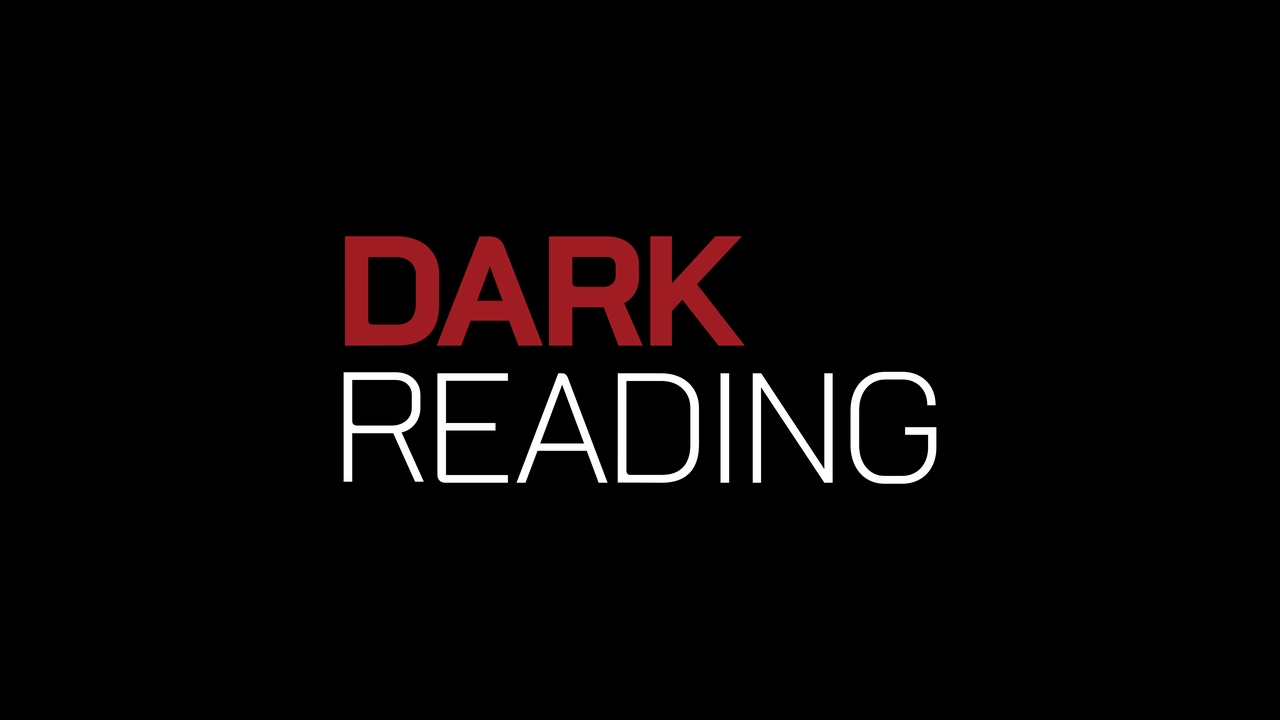
Deception — isn't that a honeypot? That's a frequently asked question when the topic of deception technology arises. This two-part post will trace the origins of honeypots, the rationale behind them, and what factors ultimately hampered their wide-scale adoption. The second post focuses on what makes up modern-day deception technology, how the application of deception technology has evolved, and which features and functions are driving its adoption and global deployment.
Almost 15 years ago, Honeyd was introduced as the first commercially available honeypot and offered simple network emulation tools designed to detect attackers. The concept was intriguing but never gained much traction outside of organizations with highly skilled staff and for research. The idea was to place a honeypot outside the network, wait for inbound network connections, and see if an attacker would engage with the decoy.
Today's attackers are more sophisticated, well-funded, and increasingly more aggressive in their attacks. Human error will continually result in mistakes for attackers to exploit. With breaches getting more severe, the population getting less patient, and the emergence of regulations and fines, in-network threat detection has become critical for every organization's security infrastructure. So much so that FBR Capital Markets forecasts that the deception technology market as a detection security control will grow to $3 billion by 2019, three times its size in 2016.
The systemic problem is that organizations are overly dependent on their prevention infrastructure, leading to a detection gap once that attacker is inside the network. For the connected world we live in, it's widely believed in the industry that it no longer works to focus only on keeping attackers out. The structure is also flawed when applied to insiders, contractors, and suppliers who have forms of privileged access. Alternatively, solutions that rely on monitoring, pattern matching, and behavioral analysis are being used as a detection control but can be prone to false positives, making them complex and resource intensive.
The concept of setting traps for attackers is re-emerging given its efficiency and the advancements in deception technology that have removed scalability, deployment, and operational functionality issues that previously had hampered the wide-scale adoption of honeypots. Consequently, companies across the financial, healthcare, technology, retail, energy, and government sectors are starting to turn to deception technology as part of their defense strategies.
Deception is still a fairly new technology, so it is not surprising that seasoned security professionals will ask, "Isn't deception just a honeypot or honeynet?" In fairness, if you consider that they are both built on trapping technology, they are similar. Both technologies were designed to confuse, mislead, and delay attackers by incorporating ambiguity and misdirecting their operations. But that is where the similarity ends.
Deception's Evolution
Gene Spafford, a leading security industry expert and professor of computer science at Purdue University, originally introduced the concept of cyber deception in 1989 when he employed "active defenses" to identify attacks that were underway, designed to slow down attackers, learn their techniques, and feed them fake data.
The next generation of advancements included low-interaction honeypots, such as Honeyd, built on limited service emulations. The ability to detect mass network scanning or automated attacks (malware, scripts, bots, scanners), track worms, and low purchase costs were the principal appeal of low-interaction honeypots. However, honeypot adoption was limited, given a number of limitations and associated management complexity, such as the following:
Honeypots were designed for detecting threats that are outside the network and were predominately focused on general research vs. responding to the more critical need for in-network detection.
Human attackers can easily figure out if a system is emulated, fingerprint it, and avoid detection from honeypots
These systems are not high interaction, limiting the attack information that could be collected and any value in improving incident response.
Attackers could abuse a compromised system and use it as a pivot point to continue their attack.
Honeypots are not designed for scalability, are operationally intensive, and require skilled security professionals to operate
Deception technology has made monumental strides in evolving from limited, static capabilities to adaptive, machine learning deception that is designed for easy operationalization and scalability. Today's deception platforms are built on the pillars of authenticity/attractiveness, scalability, ease of operations, and integrations that accelerate incident response. Based on our own internal testing and from others in the emerging deception market, deception is now so authentic that highly skilled red team penetration testers continually fall prey to deception decoys and planted credentials, further validating the technology's ability to successfully detect and confuse highly skilled cyberattackers into revealing themselves.
Related Content:
About the Author(s)
You May Also Like
CISO Perspectives: How to make AI an Accelerator, Not a Blocker
August 20, 2024Securing Your Cloud Assets
August 27, 2024