Cerber Ransomware Now Evades Machine Learning
New variant has been broken into separate harmless-looking components to fool ML-based detection systems, Trend Micro says.
March 29, 2017
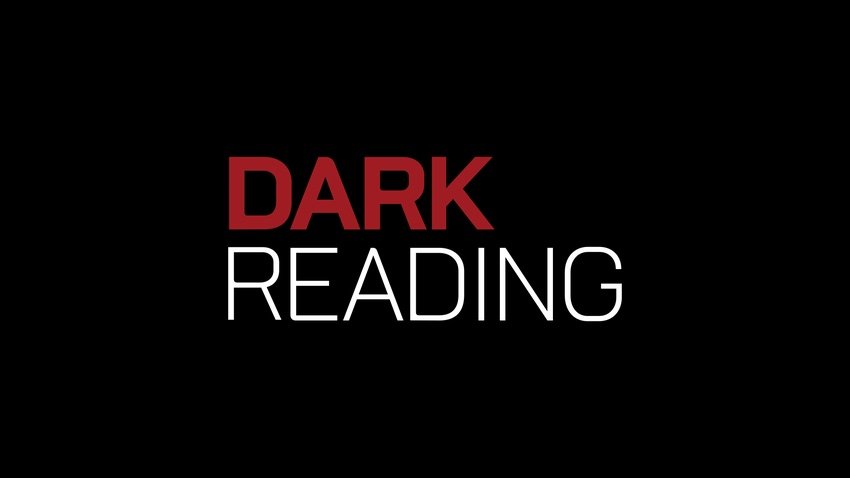
Cybercriminals have repeatedly shown an ability to innovate past whatever security controls organizations and industry have been able to throw in their way. So it is little surprise that some have begun taking a crack at machine learning tools.
Researchers at security vendor Trend Micro recently discovered a new version of the Cerber ransomware sample that appears designed specifically to evade detection by machine learning algorithms.
"The Cerber changes are really interesting as they're a direct response to changes in how some products are detecting malware," says Mark Nunnikhoven, vice president of cloud research for Trend Micro.
The newest version separates the different stages of the malware into multiple files and dynamically injects them into a running process, he says. "This helps to conceal them from various detection methods."
Like other ransomware threats, the new version of Cerber also is distributed via email. The email contains a link to self-extracting archive stored in a Dropbox account controlled by the attackers. The archive contains three files—one containing a Visual Basic script, the second a DLL, and the third, a binary file. The script is designed to load the DLL, which then reads the binary file and executes it. The binary file contains a new loader for Cerber and also the configuration settings for the malware.
The loader first checks to see if it is running in a sandbox or other protected environment. If it discerns that it's not in a protected environment, it injects the entire Cerber binary into one of several running processes, Trend Micro said in an alert this week.
"In their current form, some static machine learning-tools can have a hard time seeing the various pieces of the new configuration of Cerber," Nunnikhoven says. The malicious parts of it don't get analyzed, so the malware doesn't get flagged.
The reason is that static machine learning approaches look at the content of a file and evaluate the contents to see if they match malicious behaviors and attributes, he says.
But if the malicious content of the file is hidden for instance via encryption, or it is injected in real-time into a legitimate process, the content is not evaluated for suspicious behavior and attributes, he says.
"Say someone walks up to the door and they’ve got their hands behind their back. You look through the peephole and don’t see an immediate threat so you let them in," he says. You don't know until they are already in the house whether whatt they have in their hands is malicious or benign.
The latest innovations only make Cerber harder to detect via machine learning algorithms, he says. It can still be detected by other mechanisms. "The take-home message is that only using one technique to detect malware leaves you vulnerable if the criminals adapt to it."
News of Cerber’s latest tricks comes even as a new report from Carbon Black shows that many organizations remain unconvinced about the benefits of applying artificial intelligence and machine learning techniques to detect and stop cyber threats.
Nearly 75% of 410 security researchers that Carbon Black surveyed for the report describe AI-driven cybersecurity tools as being flawed, while 70% are convinced cyberattackers are capable of bypassing machine learning-based systems.
Mike Viscuso, co-founder and CTO of Carbon Black, says many current machine learning-based anti-malware tools are designed to stop attacks based on an inspection of files rather than behavior. They therefore miss the growing number of attacks that involve no malware files at all, he says.
Static, analysis-based approaches relying exclusively on files have been useful in the past. AI and ML-based tools can be useful in augmenting human decision-making and in spotting non-obvious relationships in massive volumes of security data. But they are of somewhat limited use in detecting non-malware attacks, he says.
Rather than using ML tools to look at individual files, organizations should be monitoring application and service activity, communications among processes, unauthorized requests to run applications, and changes to permission and credential levels, Vicuso says.
"If security tools are looking for just malware, they are missing an entire class of attacks that rely on native operating system tools to carry out nefarious actions. Attacks are evolving. So should [be] our defenses."
Related Content:
About the Author(s)
You May Also Like
Beyond Spam Filters and Firewalls: Preventing Business Email Compromises in the Modern Enterprise
April 30, 2024Key Findings from the State of AppSec Report 2024
May 7, 2024Is AI Identifying Threats to Your Network?
May 14, 2024Where and Why Threat Intelligence Makes Sense for Your Enterprise Security Strategy
May 15, 2024Safeguarding Political Campaigns: Defending Against Mass Phishing Attacks
May 16, 2024
Black Hat USA - August 3-8 - Learn More
August 3, 2024Cybersecurity's Hottest New Technologies: What You Need To Know
March 21, 2024