Duo security researchers compiled a massive dataset of public Twitter profiles and built a tool to scour profiles and detect the fakes.
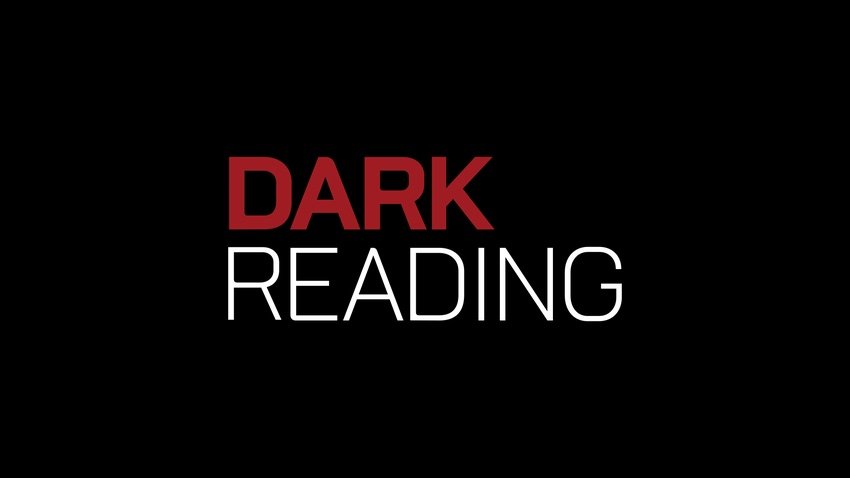
What makes Twitter bots tick? Two researchers from Duo Security wanted to find out, so they designed bot-chasing tools and techniques to separate automated accounts from real ones.
Automated Twitter profiles have made headlines for spreading malware and influencing online opinion. Earlier research has dug into the process of creating Twitter datasets and finding potential bots, but none has discussed how researchers can find automated accounts on their own.
Duo's Olabode Anise, data scientist, and Jordan Wright, principal R&D engineer, began their project to learn about how they could pinpoint characteristics of Twitter bots regardless of whether they were harmful. Hackers of all intentions can build bots and use them on Twitter.
The goal was to create a means of differentiating legitimate from automated accounts and detail the process so other researchers can replicate it. They'll present their tactics and findings this week at Black Hat in a session entitled "Don't @ Me: Hunting Twitter Bots at Scale."
Anise and Weight began by compiling and analyzing 88 million Twitter accounts and their usernames, tweet count, followers/following counts, avatar, and description, all of which would serve as a massive dataset in which they could hunt for bots. The data dates from May to July 2018 and was pulled via the Twitter API used to access public data, Wright explains.
"We wanted to make sure we were playing by the rules," Wright notes, since doing otherwise would compromise other researchers' ability to build on their work using the same method. "We're not trying to go around the API and go around limits and tools in place to get more data."
Once they obtained a dataset, the researchers created a "classifier," which detected bots in their massive pool of information by hunting for traits specific to bot accounts. But first they had to determine the details and behaviors that set bots apart.
What Makes Bots Bots?
Indeed, one of the researchers' goals was to learn the key traits of bot accounts, how they are controlled, and how they connect. "The thing about bot accounts is they can come up with identifying characteristics," Anise explains. Traits may change depending on the operator.
Bot accounts are hyperactive: Their likes and retweets are constant throughout the day and into the night. They reply to tweets quickly, Wright says. If a tweet has more than 30 replies within a few seconds, they can deduce bot activity is to blame. An account's number of followers and following can also indicate bot activity depending on when the account was created. If a profile is fairly new and has tens of thousands of followers, it's another suspicious sign.
In their research, Anise and Wright came up with 20 of these defining traits, which also included the number of unique accounts being retweeted, number of tweets with the same content per data, number of daily tweets relative to account age, percentage of retweets with URLs, ratio of tweets with photos vs. text only, number of hashtags per tweet, and distance between geolocated tweets.
Hunting Bots on the Web
The researchers' classifier tool dug through the data and leveraged these filters to detect automated accounts. Once they found initial sets of bots, they took further steps to determine whether the bots were isolated or part of a larger botnet controlled by a single operator.
"We could still use very straightforward characteristics to accurately find new bots," Wright says. "Bots at a larger scale, in general, are using many of the same techniques they have in the past few years." Some bots evolve more quickly than others depending on the operator's goals.
Their tool may have been accurate for this dataset, but Anise says many bot accounts are subtly disguised. Oftentimes accounts appeared to be normal but displayed botlike attributes.
In May, for example, the pair found a cryptocurrency botnet made up of automated accounts, which spoofed legitimate Twitter accounts to spread a giveaway scam. Spoofed accounts had randomly generated usernames and copied legitimate users' photos. They spread spam by replying to real tweets posted by real users, inviting them to join a cryptocurrency giveaway.
The botnet, like many of its kind, used several methods to evade detection. Oftentimes, malicious bots spoof celebrities and high-profile accounts as well as cryptocurrency accounts, edit profile photos to avoid image detection, and use screen names that are typos of real ones. This one went on to impersonate Elon Musk and news organizations such as CNN and Wired.
Joining the Bot Hunters
Anise and Wright are open-sourcing the tools and techniques they used to conduct their research in an effort to help other researchers build on their work and create new methodologies to identify malicious Twitter bots.
"It's a really complex problem," Anise adds. They want to map out their strategy and show how other people can use their work to continue mapping bots and botnet structures.
Related Content:
Read more about:
Black Hat NewsAbout the Author(s)
You May Also Like
Beyond Spam Filters and Firewalls: Preventing Business Email Compromises in the Modern Enterprise
April 30, 2024Key Findings from the State of AppSec Report 2024
May 7, 2024Is AI Identifying Threats to Your Network?
May 14, 2024Where and Why Threat Intelligence Makes Sense for Your Enterprise Security Strategy
May 15, 2024Safeguarding Political Campaigns: Defending Against Mass Phishing Attacks
May 16, 2024
Black Hat USA - August 3-8 - Learn More
August 3, 2024Cybersecurity's Hottest New Technologies: What You Need To Know
March 21, 2024