Extreme Makeover: AI & Network Cybersecurity
In the future, artificial intelligence will constantly adapt to the growing attack surface. Today, we are still connecting the dots.
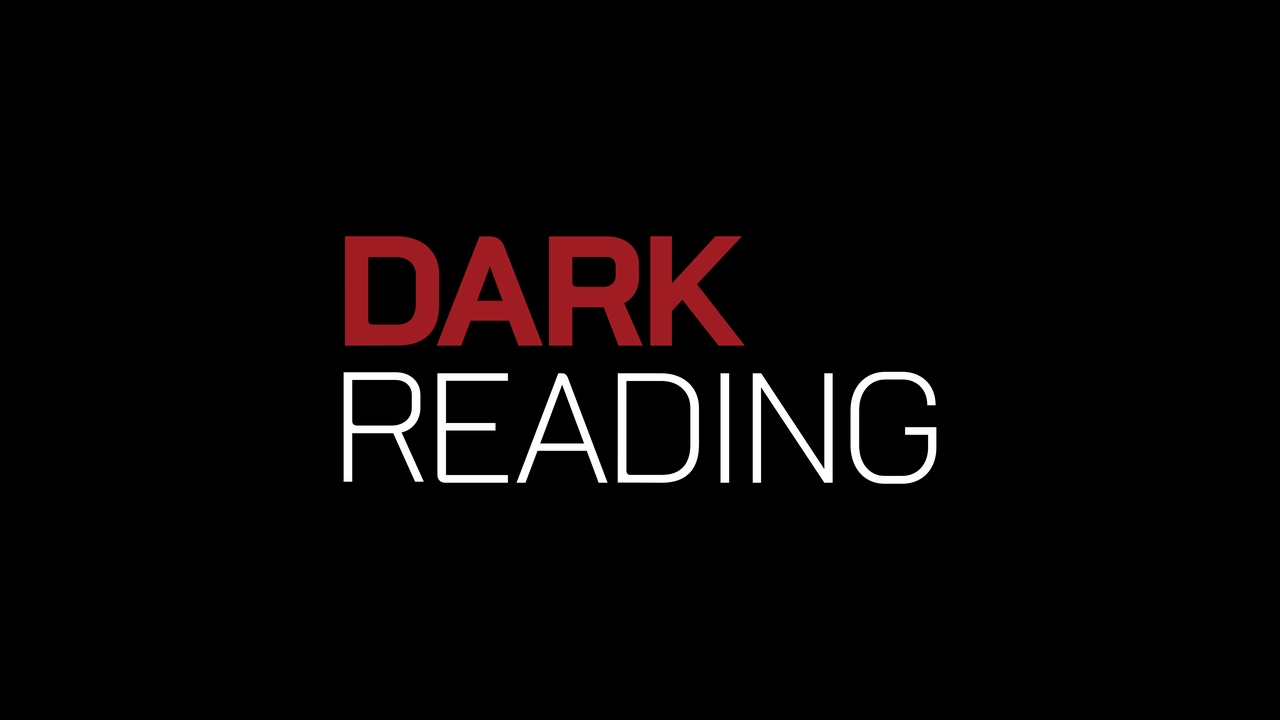
Traditional network architectures are incapable of meeting the demands of new digital paradigms involving enormous volumes of raw and processed data moving between our devices, our work, community and home networks. In this two-part series, we explore how next-gen network technology can use artificial intelligence and machine learning to become more flexible and automated.
Security strategies need to undergo a radical evolution. Tomorrow’s security devices will need to see and interoperate with each other to recognize changes in the networked environment, anticipate new risks and automatically update and enforce policies. The devices must be able to monitor and share critical information and synchronize responses to detected threats.
Sound futuristic? Not really. One nascent technology that has been getting a lot of attention recently lays the foundation for such an automated approach. It’s called Intent-Based Network Security (IBNS). IBNS provides pervasive visibility across the entire distributed network, and enables integrated security solutions to automatically adapt to changing network configurations and shifting business needs with a synchronized response to threats.
These solutions can also dynamically partition network segments, isolate affected devices and remove malware. New security measures and countermeasures can also be provisioned or updated automatically as new devices, workloads, and services are deployed or moved from anywhere to anywhere in the network, from endpoints to the cloud. Tightly integrated and automated security enables a comprehensive threat response far greater than the sum of the individual security solutions protecting the network.
Artificial intelligence and machine learning are becoming significant allies in cybersecurity. Machine learning will be bolstered by data-heavy Internet of Things devices and predictive applications to help safeguard the network. But securing these “things” and that data, which are ripe targets or entry points for attackers, is a challenge in its own right.
The Quality of Intelligence
One of the biggest challenges of using AI and machine learning lies in the caliber of intelligence. Cyber threat intelligence today is highly prone to false positives due to the volatile nature of the IoT. Threats can change within seconds; a machine can be clean one second, infected the next and back to clean again, full cycle, in very low latency.
Enhancing the quality of threat intelligence is critically important as IT teams pass more control to artificial intelligence to do the work that humans otherwise would do. This is a trust exercise, and therein lies the unique challenge. We as an industry cannot pass full control to machine automation, but we need to balance operational control with critical exercise that can escalate up to humans. This working relationship will truly make AI and machine learning applications for cybersecurity defense effective.
Because a cybersecurity skills gap persists, products and services must be built with greater automation to correlate threat intelligence to determine the level of risk and to automatically synchronize a coordinated response to threats. Often, by the time administrators try to tackle a problem themselves, it is often too late, causing an even bigger issue, or more work to be done. This can be handled automatically using direct intelligence sharing between detection and prevention products, or with assisted mitigation, which is a combination of people and technology working together. Automation can also allow security teams to put more time back into business-critical efforts instead of some of the more routine cybersecurity management efforts.
In the future, AI in cybersecurity will constantly adapt to the growing attack surface. Today, we are connecting the dots, sharing data and applying that data to systems. Humans are making these complex decisions, which require intelligent correlation through human intelligence. In the future, a mature AI system could be capable of making complex decisions on its own.
What is not attainable is full automation; that is, passing 100 percent of control to the machines to make all decisions at any time. Humans and machines must work together.
In part two, Artificial Intelligence: Cybersecurity Friend or Foe?, we highlight the flipside to the AI coin, and how cybercriminals are using it to build smarter malware.
Related Content:
About the Author(s)
You May Also Like
CISO Perspectives: How to make AI an Accelerator, Not a Blocker
August 20, 2024Securing Your Cloud Assets
August 27, 2024