Beating the Bullet: From Detection to Prevention
How deep learning technology acts pre-emptively to stop attackers before they cause serious damage.
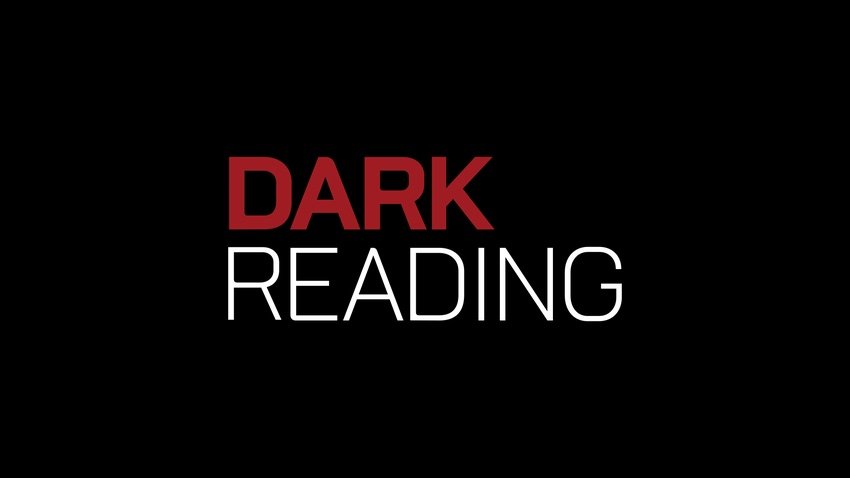
A core evaluation of artificial intelligence in cybersecurity indicates that AI is at the precipice of overhauling the attack domain. Organizations need to be ready for this next wave of attack because the reality on the ground will make it very difficult for the cybersecurity eco-system to adequately prepare themselves.
The current approach to security is that of detection and response, where the solution is triggered once a file has been accessed. In this constant pursuit of threat hunting and analysis, companies are losing the technological upper-hand against an attack landscape that is increasingly sophisticated, and where advanced attacks easily evade modern detection and response-based solutions.
Not surprisingly, CISO’s and company boards are growing weary of spending a lot of money on a raft of security products, only to later spend much more in the aftermath of a breach which inevitably occurs. This comes at an enormous cost, with time and resources spent remediating the breach rather than focusing efforts on developing revenue streams. The frequency of this scenario has prompted some industry leaders to a pursue a new frontier of prevention with a pre-emptive approach that can stop an attack before any damage can be done.
Is a preventative approach realistic? Many question the possibility, but the answer from Deep Instinct, is a resounding, Yes! Worse, there is a false sense of security in the wealth of data and analytics that a detection solution provides. Real, effective security is the difference between detection and prevention.
Beating the Bullet: The Preventative Approach
For both networks and endpoints there is a widening gap between the capability of threat actors and the efficacy of detection solutions, making it harder to adequately protect a device. In the detection and response approach an attack, or the steps to carry it out, are analyzed post-execution when the SOC team has access, as the malicious activity unfolds, creating additional artefacts. However, this effectively puts the security solution and the attack in a race, where the solution is pursuing the attack by running behind the threat actor. This reactive approach means that organizations have all the data they could possibly want about a breach, but little to actually stop it, relying mostly on human skill to identify, contain and remediate damage.
This common approach of detection and response, which is intended to reduce risk, actually exacerbates it, and highlights the business case for a pre-emptive cybersecurity solution. CISO’s shouldn’t resign themselves to solutions that operate post-execution, but should demand a solution that acts pre-emptively to keep them protected.
By definition, a zero-time preventative solution incorporates five elements to distinguish it from a detection and response-based solution, or other supposedly preventative tools. These include:
1. Pre-execution – The solution is designed to be triggered before any malicious business logic takes place. For example, as soon as a file is accessed, downloaded on to a device, or malicious code injection is fully executed.
2. Autonomous – Once the solution is activated, it autonomously analyzes and makes decisions on prevention and alerts, regardless of human involvement and Internet connectivity. If a human is involved it’s not a real-time solution.
3. Zero-time – Any new data artefact or file must be analyzed in a matter of milliseconds, prior to being executed, opened or causing compromise, effectively providing a zero-time response.
4. All threats – The solution’s design should cover a broad range of cyberattack vectors and surfaces, both known and yet unknown threats.
5. All environments – the solution should protect a wide range of OSes and environments, be it networks, endpoints, mobile devices or servers, from a single unified platform.
Currently, deep learning is the only technology available that is able to deliver these five elements to provide a real prevention-oriented solution. The adaptation and application of deep learning makes it possible to harness its innate advantages of fast inference and high accuracy to provide prevention. The rigorous analysis of deep learning also provides a remarkably low false positive rate, despite the higher rates of detected files.
To learn more about solutions that work in pre-execution, read the full article.
About The Author
Guy Caspi, CEO & Co-founder, Deep Instinct
A serial entrepreneur, Guy Caspi has spearheaded companies in senior positions through entire life cycles, from start up, accelerate growth and up to IPO in Nasdaq. Guy has in-depth knowledge of machine learning and deep learning assimilation in cybersecurity, which he has applied to his unique go-to-market execution experience.
About the Author(s)
You May Also Like
Beyond Spam Filters and Firewalls: Preventing Business Email Compromises in the Modern Enterprise
April 30, 2024Key Findings from the State of AppSec Report 2024
May 7, 2024Is AI Identifying Threats to Your Network?
May 14, 2024Where and Why Threat Intelligence Makes Sense for Your Enterprise Security Strategy
May 15, 2024Safeguarding Political Campaigns: Defending Against Mass Phishing Attacks
May 16, 2024
Black Hat USA - August 3-8 - Learn More
August 3, 2024Cybersecurity's Hottest New Technologies: What You Need To Know
March 21, 2024