Advanced Analytics + Frictionless Security: What CISOS Need to Know
Advances in analytics technologies promise to make identity management smarter and more transparent to users. But the process is neither straightforward nor easy. Here's why.
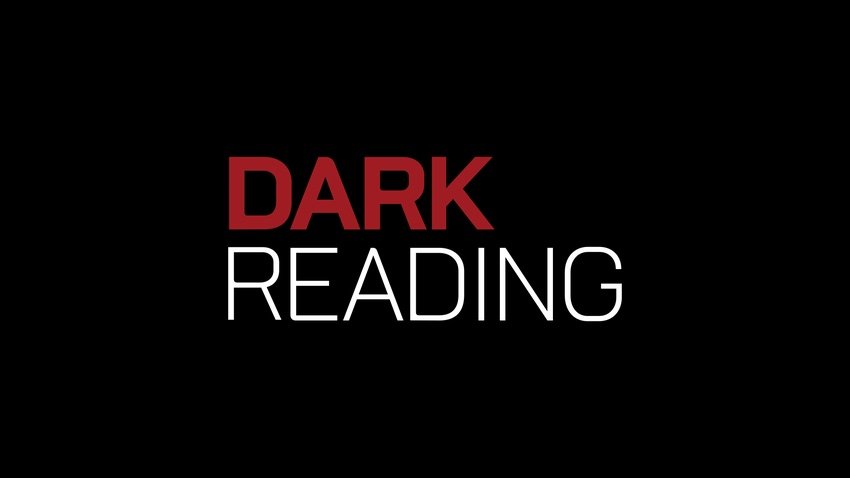
The digital transformation of business processes is forcing CISOs to implement security processes that move at customer speed and reduce friction. This is placing greater strain on access management, because organizations need to protect themselves from account compromise and other digital threats while simultaneously providing a better user experience.
An approach known as adaptive access management can support run-time use cases that address CISOs' security needs while reducing speed bumps for the CIO. Today, advances in analytics involving multiple vendors and technologies are providing the foundation to make this possible by enabling real-time automated decision-making that doesn't require human intervention.
For example, an organization could monitor user access and activity in real time to capture and forward attributes, such as how a person holds his or her phone, device configuration, or apps used most frequently, into a risk engine. As described in a recent Wall Street Journal post, machine learning analytics create an individual risk score for each user. When actions deviate significantly from each user's baseline normal behavior, the risk score is increased. When risk thresholds are exceeded, the app may restrict access to certain functions or request another form of authentication before allowing the user to proceed.
Traditional approaches to adaptive access control were based on static roles and rules. These were created and maintained by security administrators, which resulted in a lag between a threat being identified and when a new rule was deployed. The emergence of machine learning techniques produces greater automation since more factors can be used to detect new threats with less human effort and reduced time frames.
Deploying adaptive access management and automated security responses that are dramatically smarter and more agile is neither straightforward nor easy. Let's consider six different implementations of analytics that are required to reduce security "friction."
Implementation 1: Risk Scoring
Adaptive access management requires a large number of factors be assessed together rather than individually. This is important because high risk in one factor can be compensated by another. Let's say a company's business partner makes an access request from a country where they don't operate. This may indicate a high risk. However, an access request from a longtime business partner in a fast-growing company that is opening up a new office in a new country may be low-risk. Under these circumstances, the country risk is contextual, not absolute. Making sophisticated access management decisions requires using an overall risk score to mediate conflicts.
Implementation 2: Behavior Analytics
By ingesting and monitoring activity data (typically logs from different sources) of a user's behavior, and following several weeks of training, behavior analytics can determine in real time whether an access request is normal. This form of analytics can identify when a user's credentials have been compromised, so access can be revoked in real time before damage occurs.
Implementation 3: Anomaly Detection
Analytics that use machine learning can identify when actions deviate from what is normal or expected. Traditionally, anomaly detection processes have created large numbers of false positives. Advanced analytics, meanwhile, can greatly reduce these.
Implementation 4: Dynamic Peer-Group Analysis
Analytics and machine learning can generate and use dynamically generated peer groups to further refine the analysis of what is normal and abnormal behavior to reduce false positives. If a new group member performs a sensitive action for the first time, it might be flagged as high risk. However, if other group members regularly perform the action, then it would not be considered high risk, even if it represents an anomaly for that specific user.
Implementation 5: Continuous Monitoring
The use of analytics enables more actions to be monitored, analyzed, and acted upon without long delays and a lot of false positives. This makes it possible to both evaluate risk at the initial time that access is requested and continue to monitor it for the entire length of a session. If an authorized user, for example, accesses an application and leaves to get coffee, this valid session could be hijacked.
Implementation 6: Predictive Analytics
Analytics can also be used to predict future events and recommend how an access management system should operate. For example, predictive analytics could determine that an authentication attempt from an IP address associated with past fraud events will likely be involved in new fraud attempts. The session could be flagged as higher risk for closer monitoring, or if other risk factors were present, be terminated.
Advances in analytics promise to make security smarter and more transparent to users. The challenge for CISOs is stitching together the systems needed to both gather the big data to make analytics-based decisions and implement the appropriate adaptive responses.
Join Dark Reading LIVE for two days of practical cyber defense discussions. Learn from the industry’s most knowledgeable IT security experts. Check out the INsecurity agenda here.
About the Author(s)
You May Also Like
Beyond Spam Filters and Firewalls: Preventing Business Email Compromises in the Modern Enterprise
April 30, 2024Key Findings from the State of AppSec Report 2024
May 7, 2024Is AI Identifying Threats to Your Network?
May 14, 2024Where and Why Threat Intelligence Makes Sense for Your Enterprise Security Strategy
May 15, 2024Safeguarding Political Campaigns: Defending Against Mass Phishing Attacks
May 16, 2024
Black Hat USA - August 3-8 - Learn More
August 3, 2024Cybersecurity's Hottest New Technologies: What You Need To Know
March 21, 2024