The Containerization of Artificial Intelligence
AI automates repetitive tasks and alleviates mundane functions that often haunt decision makers. But it's still not a sure substitute for security best practices.
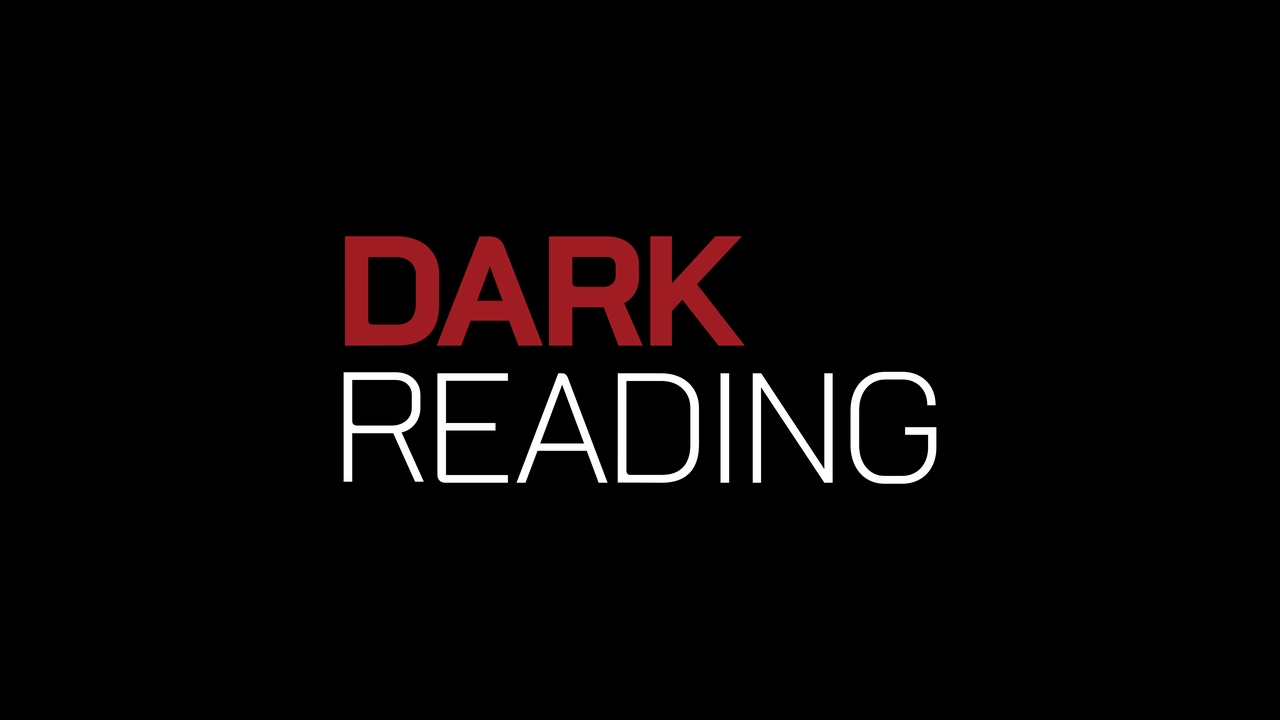
Artificial intelligence (AI) holds the promise of transforming both static and dynamic security measures to drastically reduce organizational risk exposure. Turning security policies into operational code is a daunting challenge facing agile DevOps today. In the face of constantly evolving attack tools, building a preventative defense requires a large set of contextual data such as historic actuals as well as predictive analytics and advanced modeling. Even if such feat is accomplished, SecOps still needs a reactive, near real-time response based on live threat intelligence to augment it.
While AI is more hype than reality today, machine intelligence — also referred to as predictive machine learning — driven by a meta-analysis of large data sets that uses correlations and statistics, provides practical measures to reduce the need for human interference in policy decision-making.
A typical by-product of such application is the creation of models of behavior that can be shared across policy stores for baselining or policy modifications. The impact goes beyond SecOps and can provide the impetus for integration within broader DevOps. Adoption of AI can be disruptive to organizational processes and must sometimes be weighed in the context of dismantling analytics and rule-based models.
The application of AI must be constructed on the principle of shared security responsibility; based on this model, both technologists and organizational leaders (CSOs, CTOs, CIOs) will accept joint responsibility for securing the data and corporate assets because security is no longer strictly the domain of specialists and affects both operational and business fundamentals. The specter of draconian regulatory compliance such as fines articulated by the EU's General Data Protection Regulation provides an evocative forcing function.
Focus on Specifics
Instead of perceiving AI as a cure-all remedy, organizations should focus on specific areas where AI holds the promise of greater effectiveness. There are specific use cases that provide a more fertile ground for the deployment and evolution of AI: rapid expansion of cloud computing, microsegmentation, and containers offer good examples. Even in these categories, shared owners must balance the promises and perils of deploying AI by recognizing the complexity of technology while avoiding the cost of totally ignoring it.
East-west and north-south architecture of data flow has its perils as we witnessed in the recent near-meltdown of public cloud services. The historic emphasis on capacity and scaling has brought us to clever model of computing which involves many layers of abstraction. With abstraction, we have essentially removed the classic stack model and therefore adding security to it presents a serious challenge.
Furthermore, the focus away from the nuts and bolts of infrastructure to application development in isolation and insulation has given birth to the expectation that even geo-scale applications inside containers and Web-scale micro services can be independently secured while maintaining an automated and scalable middleware. Hyperscale computing, relying on millisecond availability in distributed zones, is more than an infrastructure play and increasingly relies on microsegmentation and container-based application services — a phenomenon whose long-term success depends on AI.
In the '90s, VLANs were supposed to give us protective isolation and the ability to offer a productive computing space based on roles and responsibilities. That promise had fallen far short of expectations. Microsegmentation and containers are in a way a post-computing evolution of VLANs. They have brought other benefits such as reducing pressure on firewall rules; no longer there is a need to keep track of exponentially growing rules with little visibility in situations that lead to false positives and false negatives. Although the overall attack surface is reduced, and collateral damage is partially abated, the potential for more persistent breaches are not reduced. AI tools can zero in on a smaller subset of data and create better mapping without affecting the user productivity or undermining the overlay concept of segmented computing.
It is pretty much a one-two-three punch: the organization can look at all available metadata, feed that to the AI, and then take the output of AI to predictive analytics engines and create advanced modeling of potential attacks that are either in progress or will soon commence. We are still a few years away from the implementation of another potential step: machine-to-machine learning and security measures whereby machines can observe and absorb relevant data and modify their posture to protect themselves from predicted attacks.
AI can also provide substantial value in other emerging areas such as autonomous driving. Cars are increasingly resembling computing machines with direct cloud command and control. From offline modeling based on fuzzing to real-time analysis of sensor data, we may rely on AI to reduce risks and liabilities.
Artificial intelligence is not a panacea; however, it automates repetitive tasks and alleviates mundane functions that often haunt security decision makers. Like other innovations in security, it will go through its evolutionary cycle and eventually finds its rightful place. In the meantime, there is still no sure substitute for security best practices.
Related Content:
Black Hat Asia returns to Singapore with hands-on technical Trainings, cutting-edge Briefings, Arsenal open-source tool demonstrations, top-tier solutions and service providers in the Business Hall. Click for information on the conference and to register.
About the Author(s)
You May Also Like
CISO Perspectives: How to make AI an Accelerator, Not a Blocker
August 20, 2024Securing Your Cloud Assets
August 27, 2024