Security Analytics: Making the Leap from Data Lake to Meaningful Insight
Once you've got a lake full of data, it's essential that your analysis isn't left stranded on the shore.
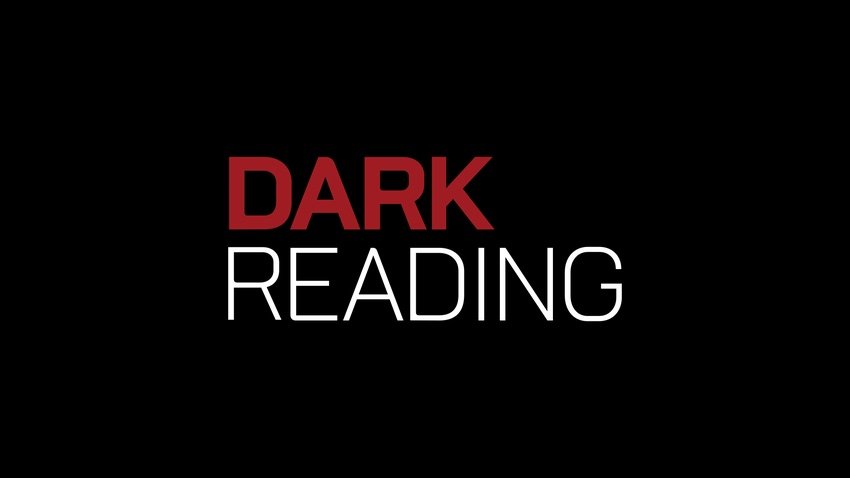
Second of a two-part series.
Lots of technology and security teams, particularly in finance, are running data lake projects to advance their data analytics capabilities. Their goal is to extract meaningful, timely insights from data, so that security leaders, control managers, IT, and security operations can make effective decisions using information from their live environment.
During the four phases of a data lake project (build data lake; ingest data; do analysis; deliver insight), the hurdles to success are different. In the first two phases, it's easy for a data lake to become a data swamp. In the last two, it's easy to have a lake full of data that delivers a poor return on investment. Here are three steps to avoid that happening.
Step 1: Clean up messy analysis workflows from the get-go.
Security teams know the frustrations of ad hoc data analysis efforts only too well. For example, a risk question from an executive is given to an analyst, who collects data from whatever parts of the technology "frankenstack" he or she can get access to. This ends up in spreadsheets and visualization tools. Then, after days or weeks of battling with hard-to-link, unwieldy data sets, the results of best-effort analysis are sent off in a slide deck. If this doesn't answer the questions "So what?" and "What now?" the cycle repeats.
Automating the process of putting data from the many and varied security-relevant technologies that exist in an enterprise environment into a data lake, and then just replicating a process like the one above, means analysis efforts may start sooner. However, if data isn't structured so it's easy to understand and interact with, it doesn't get easier to deliver meaningful output.
To avoid this, look at ways to optimize your data analysis workflow. Consider everything from how you ingest, store, and model data to how you scope questions with stakeholders and set their expectations about "speed to answer." Build a framework for iterating analysis — and be clinical about quickly proving or disproving the ROI a data set can contribute toward the stakeholder-requested insight. Also think about how to build a knowledge base about what relationships in what data sets are valuable, and which aren't. It's important that analysts' experience isn't trapped in their heads and that teams can avoid repeating analysis that eats up time and money without delivering value.
Step 2: Buy time for the hard work that needs doing up front.
There's often a lot of complexity involved in correlating data sets from different technologies that secure an end-to-end business process. When data analytics teams first start working with data from the diverse security and IT solutions that are in place, they need to do a lot of learning to make sure the insight they present to decision makers is robust and has the necessary caveats.
This learning is on three levels: first, understanding the data; second, implementing the right analysis for the insight required; and third, finding the best way to communicate the insight to relevant stakeholders so they can make decisions.
The item with the biggest lead time is "understanding the data." Even if security teams interact regularly with a technology's user interface, getting to grips with the raw data it generates can be a hard task. Sometimes there's little documentation about how the data is structured, and it can be difficult to interpret and understand the relevance of information from just looking at the labels. As a result, data analytics teams usually need to spend a long time answering questions such as: What do the fields in each data source mean? How does technology configuration influence the data available? Across data sources, how does the format of information referring to the same thing differ? And what quirks can occur in the data that need to be accounted for?
It's critical to set expectations with budget holders and executives about how important this is and the time it will take. This isn't just because understanding and modeling data is a key enabler of delivering speed to insight in the long term. It's also because it's easy to create "data skeptics" when pressure for fast results leads to rushed analysis that delivers incorrect information.
Step 3: Plan to scale from the start for long-term success.
Providing data-driven risk and security insights for executives and operations teams usually means answering the following questions: What's our status? Is it good or bad? If it's bad, why? Do we act or gather more information? If we act, what are our best cost actions?
Once data analytics teams start providing high-value insights that answer these questions — for example, "What are our best cost options to achieve large reductions in risk due to vulnerability exposure?" — the next challenge is scaling the team's capability to answer more (and usually more difficult) questions.
At this point, the number of people on your data analytics team can become a bottleneck to servicing requests for insight. And when other departments like IT, audit, and compliance see the benefits from data-driven decisions, the volume of requests for insight can increase exponentially.
To avoid this, think about how to enable people who aren't data analytics experts to interact with data so they can "self-serve" insights. Who are your different stakeholders? What insights and metrics do they need to run their business process? How will they need to explore the dimensions of data relevant to answer questions they have and diagnose issues? Mapping a workflow of "these stakeholders, need this insight, from this data" helps answer these questions and will enable you to identify data sets that are relevant to decisions for multiple stakeholders. In turn, this helps you focus efforts to understand high-value data sets as early as possible.
Related Content:
Security Analytics: Don't Let Your Data Lake Turn Into A Data Swamp (Part 1 of 2-part series)
Learn from the industry’s most knowledgeable CISOs and IT security experts in a setting that is conducive to interaction and conversation. Click for more info and to register.
About the Author(s)
You May Also Like
Beyond Spam Filters and Firewalls: Preventing Business Email Compromises in the Modern Enterprise
April 30, 2024Key Findings from the State of AppSec Report 2024
May 7, 2024Is AI Identifying Threats to Your Network?
May 14, 2024Where and Why Threat Intelligence Makes Sense for Your Enterprise Security Strategy
May 15, 2024Safeguarding Political Campaigns: Defending Against Mass Phishing Attacks
May 16, 2024
Black Hat USA - August 3-8 - Learn More
August 3, 2024Cybersecurity's Hottest New Technologies: What You Need To Know
March 21, 2024