Machine Learning For Cybersecurity Not Cybercrime
Cybercriminals have yet to adopt machine learning for offensive attack strategies - and they probably won’t for a long time.
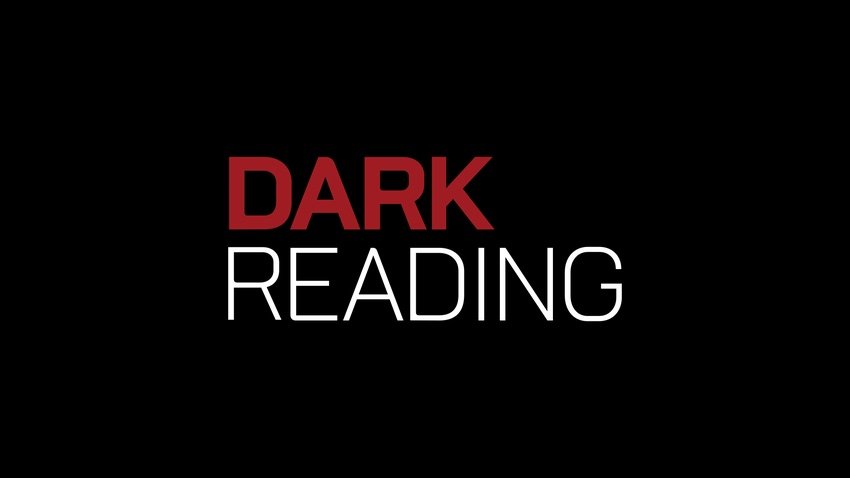
The cybersecurity industry has always been under constant strain from cybercriminals and malware. With increasing integration of hardware, software and services being built into every aspect of our lives, the task of keeping data secure has become even more difficult.
The arsenal of tools that cybercriminals now have at their disposal has raised concerns for security companies, and turned the criminals into threat actors who can create, disseminate and penetrate a target’s defenses using custom-built and never-before-seen malware. The security industry has had to adopt a new way of dealing with the unknown by leveraging the powerful capabilities of machine learning algorithms.
Cybersecurity & Machine Learning
Because targeted and advanced threats that seek to prey on organizations and businesses often evade traditional security mechanisms, machine learning algorithms have stepped in to fill in the gap between proactivity and detection. While humans are great at in-depth analysis and pinpointing code subtleties in malicious samples, machine learning is better at applying models on large data without tiring or complaining of repetitive tasks.
In the context of big data – where everything connected to the Internet from IoT devices to physical and virtual endpoints is a potential source of information or point of attack - machine learning can be trained to parse, analyze and interpret that data with little no effort.
The human component, however, is responsible for the accuracy of the machine learning model and for supplying its “wits.” Cybersecurity specialists with years of experience in reverse engineering malware samples and analyzing attack techniques are the ones who usually transfer their experience to machine learning algorithms, training the algorithms for behavior analytics and anomaly detection. While machine learning algorithms range from neural networks to genetic algorithms, their ultimate goal is to adapt to variations of a baseline behavior.
Do Cybercriminals Use Machine Learning?
No, they don’t! That’s because they already have a wide range of tools and mechanisms that have automated not only malware development but also ensured that each new malware sample is unique.
Obfuscation and polymorphism are just two examples cybercriminals use to create and deliver ransomware samples to both average users and organizations. They are so effective that ransomware is estimated to have inflicted at least $1 billion in financial losses in 2016 alone.
Encryption is another powerful tool consistently leveraged by cybercriminals to mask data exfiltration and even extort victims. The whole point of the cybercrime industry is to constantly create new packing mechanisms for malware samples, and not necessarily come up with innovative attack techniques or behavior. This doesn’t require machine learning; it involves constant algorithm tweaking or the development of obfuscation functions or encryption algorithms.
Is Machine Learning Offensive or Defensive?
When applied in the “cyber” context, current machine learning capabilities are mostly defensive. Machine learning helps the security industry tackle more than 500 million malware samples. Cybercriminals have yet to adopt machine learning and they probably won’t for a long time.
While there have been examples of machine learning algorithms being pitted against each other; one looking for software vulnerabilities and the other trying to patch them – these exercises were for demonstration only.
Of course, machine learning can be considered to have offensive capabilities when applied in the gaming industry, as it can be trained to take out virtual foes with the same accuracy as their human counterparts. However, they’re yet to be used for cybercriminal activities.
About the Author(s)
You May Also Like
Beyond Spam Filters and Firewalls: Preventing Business Email Compromises in the Modern Enterprise
April 30, 2024Key Findings from the State of AppSec Report 2024
May 7, 2024Is AI Identifying Threats to Your Network?
May 14, 2024Where and Why Threat Intelligence Makes Sense for Your Enterprise Security Strategy
May 15, 2024Safeguarding Political Campaigns: Defending Against Mass Phishing Attacks
May 16, 2024
Black Hat USA - August 3-8 - Learn More
August 3, 2024Cybersecurity's Hottest New Technologies: What You Need To Know
March 21, 2024