Knowledge Gap Series: The Myths Of Analytics
It may not be rocket science, but it is data science.
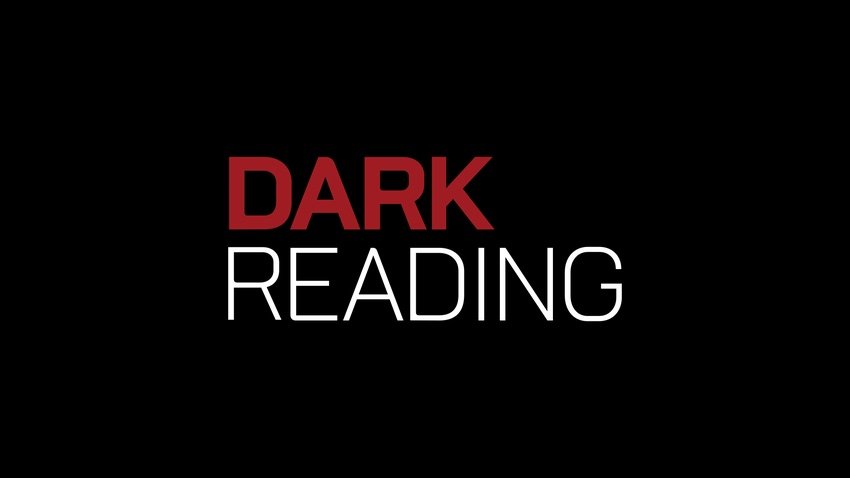
Do you have your eye on machine learning or a nice neural network to help your security team make decisions faster? Be aware that there are quite a few myths circulating about how these work; even the language used can be confusing. Many new terms -- and some familiar words --have different meanings in the world of statistical analytics. For example, “variable” means something significantly different to a programmer than to a statistician. And the capabilities of a statistician are different from those of a data scientist.
Let’s start with building an analytical model. This does not happen quickly, because you need to capture enough data from your environment to give you a representative distribution. Roughly put, the distribution is the shape of the data (much like the classic bell curve from college), including the upper and lower limits, symmetry, presence of outliers, and other characteristics. There are dozens of statistical distributions, and the choice is critical because they form the foundation of the behavioral model. Another issue is cleaning the data prior to exploring potential models. How do you want to deal with outliers? What weights will you assign to the various components? Which ones are fully or partially dependent?
Some machine-learning technologies will gather and analyze the data to try and determine an appropriate distribution for you, but you still need to be able to understand the decision. For example, many data sets do not fit the symmetry of a bell curve (formally called a normal distribution), and the distribution that fits probably has an unfamiliar name. Some of these tools only work with certain types of data sets, and all of them have underlying assumptions that you need to understand. You also need to understand some of the math, at least at a cursory level. Different tools may use different equations for a similar application -- such as correlation coefficients that show the degree of dependence between two sets of data, especially if the relationships are nonlinear.
Say you have been through this exercise, some statisticians and data scientists have advised you, and you now have an analytical model for identifying data exfiltration, phishing attacks, or some other security event. What is the appropriate level of confidence in the results? No model is always right, and you need to know how well the model fits, what your statistical level of confidence is, and what to look for when an automated decision gets punted for human judgment. These models are ultimately built by humans, so you also need to make sure that you have an appropriate level of trust in the quality and ethics of your modeller.
Statistics, analytics, and machine learning are powerful tools that will help resolve security problems faster, with fewer resources. They will empower the next wave of automated and even predictive defenses. However, this will take time, and we have to work our way up from reactive models, through proactive ones, before we get to predictive.
This journey is going to require some learning on your part, whether it is a review of your college stats classes or building an understanding of the terms and concepts, so that you can communicate clearly and effectively with the statisticians and data scientists that will be joining your team. You need to ensure that your data scientists have a strong working knowledge of statistics, as this title is loosely defined and may be overused. Finally, you will need to be able to translate these concepts and plans to members of the C-suite, who may be skeptical about the uses and abuses of statistics.
My intent is not to scare you off with the amount of work involved. When properly implemented, the security benefits of big data analytics are substantial.
The Intel Security Knowledge Gap series brings forward unique educational content to bridge the gap between what cybersecurity professionals know and what they need to know to be successful against the threat landscape of today and tomorrow.
About the Author(s)
You May Also Like
Beyond Spam Filters and Firewalls: Preventing Business Email Compromises in the Modern Enterprise
April 30, 2024Key Findings from the State of AppSec Report 2024
May 7, 2024Is AI Identifying Threats to Your Network?
May 14, 2024Where and Why Threat Intelligence Makes Sense for Your Enterprise Security Strategy
May 15, 2024Safeguarding Political Campaigns: Defending Against Mass Phishing Attacks
May 16, 2024
Black Hat USA - August 3-8 - Learn More
August 3, 2024Cybersecurity's Hottest New Technologies: What You Need To Know
March 21, 2024