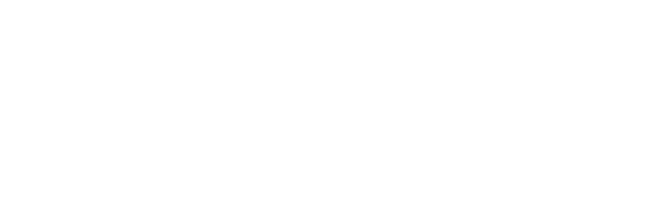
News, news analysis, and commentary on the latest trends in cybersecurity technology.
How AI/ML Can Thwart DDoS Attacks
When properly designed and trained, artificial intelligence and machine learning can help improve the accuracy of distributed denial-of-service detection and mitigation.
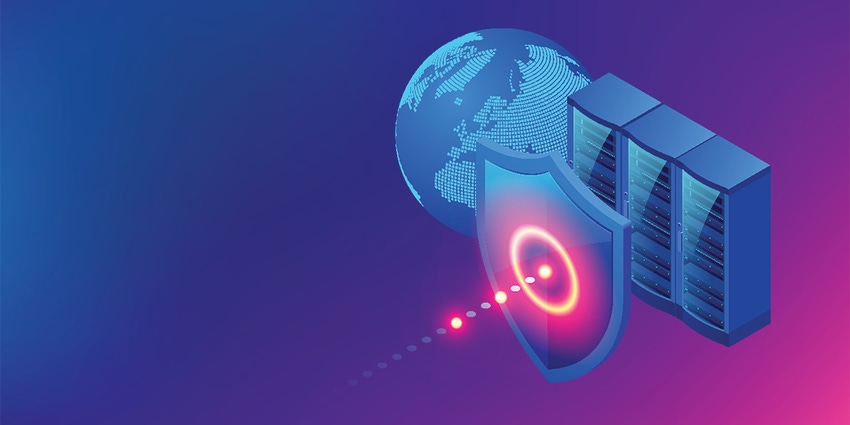
After early excitement about artificial intelligence (AI) in the late 1980s and early 1990s, followed by a couple of "AI winters" — periods of reduced funding, interest and even disillusionment — we now again see great enthusiasm about all things related to AI and machine learning (ML). It is no wonder that AI/ML is also being considered for network security, including distributed denial-of-service (DDoS) protection.
It's not that AI/ML algorithms have changed so radically — but they have matured. In network security, like in many other fields, the abundance of data and greater-than-ever processing power makes it feasible to implement new AI/ML algorithms in silicon or in the cloud, allowing us to teach machines to be more accurate and faster than humans are.
With DDoS security, the problem is distinguishing "good" from "bad" traffic and minimizing the mitigative actions to reduce the effect on "good" traffic. Apart from accuracy and speed, the percentage of false positives indicates how good your detection is — the lower, the better. Until recently, the industry-accepted rate of 5% to 10% false positives meant that neutralizing a 2Tbps-size DDoS attack could also block 100Gbps to 200Gbps of legitimate network traffic. This needs to improve by at least an order of magnitude.
AI/ML for Better DDoS Detection
AI/ML can help network security teams make more accurate and faster decisions about what constitutes a DDoS threat or is an ongoing attack. Knowing the larger Internet-security context is essential — a global perspective of traffic down to the IP address level, with prior history of traffic patterns and abuse — can help prevent making a snap decision about whether certain traffic flows are legitimate. It's like a credit card company tracking all transactions in order to decide which ones are fraudulent.
Big data collected from the network itself — in the form of telemetry from IP routers, enhanced with the larger security context — provides a great base for training AI/ML models to recognize DDoS patterns. Still, human intelligence is irreplaceable: People need to teach AI/ML what to look for. And there is a lot to be taught, from recognizing a botnet DDoS (coming from thousands of IoT devices as regular IP traffic) to understanding when seemingly separate network patterns are all parts of a larger, coordinated DDoS activity.
Better Mitigation, Too
Another important role for AI/ML is in defining DDoS mitigation strategies and driving real-time tactics based on changing network conditions and mitigation results.
DDoS detection is a big data problem with somewhat unconstrained resources, limited only by the processing platforms. DDoS mitigation, however, is a problem where resources are constrained. Mitigation capabilities, capacity, and scale can differ from product to product and from one network to another. The actual mitigative actions need to consider all of those details and more — preferences about the number of security filters applied on routers, whether NETCONF or Flowspec should be used, etc. All of these constraints can be passed to an AI/ML system to drive networkwide AI/ML-optimized mitigation.
Additionally, AI/ML algorithms could be used to calculate the efficiency (as measured by false-positive rates) of suggested mitigation scenarios and to test and evaluate different what-if scenarios offline to improve the mitigation further.
Understanding Wider Context Is Key
Big data platforms can efficiently sift through massive amounts of data, ingesting information about the Internet-wide security-related context and real-time network data about network flows, usage patterns, and other relevant metrics.
With the help of AI/ML algorithms, it is now possible to detect DDoS activity early and take immediate, targeted, and optimized mitigation measures to thwart such attacks.
By incorporating big data analytics and AI/ML into all stages of a comprehensive DDoS security strategy, we can guard our networks against malicious DDoS attacks, keep the services running, and protect users online.
About the Author(s)
You May Also Like
Key Findings from the State of AppSec Report 2024
May 7, 2024Is AI Identifying Threats to Your Network?
May 14, 2024Where and Why Threat Intelligence Makes Sense for Your Enterprise Security Strategy
May 15, 2024Safeguarding Political Campaigns: Defending Against Mass Phishing Attacks
May 16, 2024Why Effective Asset Management is Critical to Enterprise Cybersecurity
May 21, 2024
Black Hat USA - August 3-8 - Learn More
August 3, 2024Cybersecurity's Hottest New Technologies: What You Need To Know
March 21, 2024