A Real World Analogy For Patterns of Attack
Patterns reveal exponentially more relevant information about attempted malfeasance than singular indicators of an attack ever could.
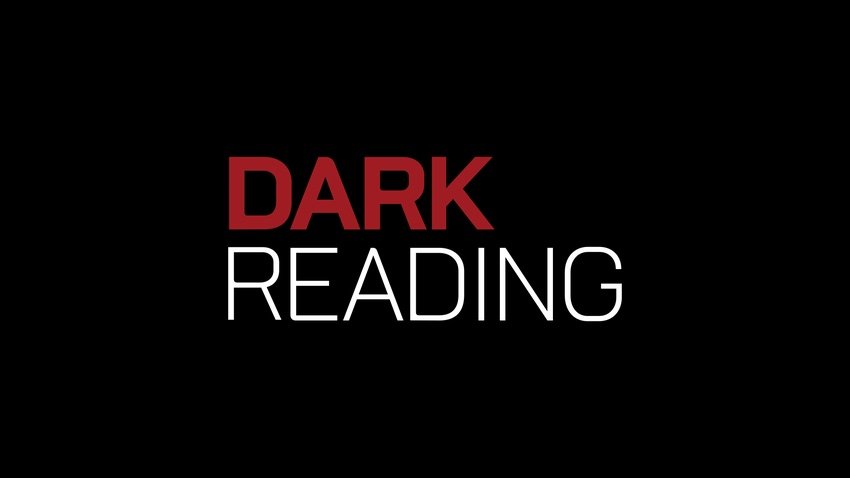
In last week’s post, we talked about the important differences between indicators of compromise (IOCs) and patterns of attack (POAs). To better understand why patterns of attack are exponentially better, consider this physical-world analogy.
Convenience Store Robbery
Investigating Using IOCs: Investigators come to find that during this robbery, the criminal used a crowbar to break the glass on the front door; wore a blue shirt; had short, light-colored hair; and used a hiking backpack to stash the cash from the register.
What exactly have the investigators learned, if anything?
Crowbars are sometimes used in smash-and-grab robberies.
“Ok, let’s make sure to look out for anyone carrying a crowbar in plain sight.”
Sometimes, people wearing blue shirts with short, light-colored hair may commit crimes.
“Ok, let’s look out for anyone wearing a blue shirt that has light-colored hair.”
Hiking backpacks are sometimes a tool used during burglaries.
“Ok, let’s try to monitor hiking backpack sales in this area moving forward.”
That’s not a lot of substance to go on for this investigation. We have an incomplete picture.
Investigating the Same Crime Using POAs: Investigators come to find that for the past two weeks, someone has been parked in the store parking lot at night noting what time the clerk locks up for the night and what time the rent-a-cop security detail passes by the store. The burglar drives to the store at precisely the right time of night to break in. He knows there’s an archaic alarm system on the door so he successfully cuts power to the building prior to entering to deactivate the alarm. Once inside, he approaches the register, opens the register drawer, takes the cash and exits the store.
What patterns has the burglar exhibited here?
In order to get to the store, the burglar needs to drive to (or close to) the store’s location.
He has to deactivate the alarm.
He has to enter the building before getting access to the real goal, the cash register.
He has to open the register drawer.
He needs to leave the premises with the cash in hand.
Individually, these single indicators of an attack tell an incomplete picture. Driving to, or near, a store doesn’t reveal a whole lot to investigators. Thousands of people do that every single day. What about entering the store? Same idea. Thousands of people. And while deactivating an alarm or opening a register drawer appear to be a lot closer to “burglary-type” activity, there are numerous instances where both are done on a regular basis. These are simply indications that a crime might be committed.
It’s only when this sequence, or pattern, of attack behaviors shows up do we really start to see what is happening from an investigation standpoint.
When someone drives near the store late at night THEN attempts to enter the building THEN attempts to deactivate the alarm THEN opens the register drawer, we almost CERTAINLY have an attempted burglary on our hands.
Also notice how none of the behavior patterns exhibited can be changed. Failure to do any one of the steps will result in a failed mission for the robber. It’s ripe for disruption-in-depth, but we’ll leave that for another day.
Patterns reveal exponentially more relevant information about attempted malfeasance than singular indicators of an attack ever could. Context, relationships, and the sequence of events all matter. If you’re just looking for one item in the sequence of events, that’s when issues like too many tips or -- in the cyberworld -- false positives start becoming a bigger issue than the malicious behavior itself. After all, if you cannot respond to a tip or an alert, it’s just noise.
About the Author(s)
You May Also Like
Beyond Spam Filters and Firewalls: Preventing Business Email Compromises in the Modern Enterprise
April 30, 2024Key Findings from the State of AppSec Report 2024
May 7, 2024Is AI Identifying Threats to Your Network?
May 14, 2024Where and Why Threat Intelligence Makes Sense for Your Enterprise Security Strategy
May 15, 2024Safeguarding Political Campaigns: Defending Against Mass Phishing Attacks
May 16, 2024
Black Hat USA - August 3-8 - Learn More
August 3, 2024Cybersecurity's Hottest New Technologies: What You Need To Know
March 21, 2024