4 Practical Questions to Ask Before Investing in AI
A pragmatic, risk-based approach can help CISOs plan for an efficient, effective, and economically sound implementation of AI for cybersecurity.
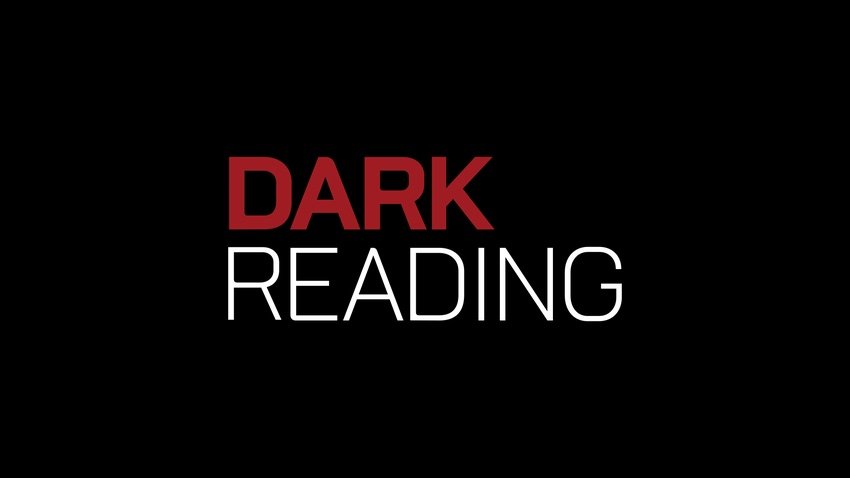
Artificial intelligence (AI) could contribute up to $15.7 trillion to the global economy by 2030, according to PwC. That's the good news. Meanwhile, Forrester has warned that cybercriminals can weaponize and exploit AI to attack businesses. And we've all seen the worrisome headlines about how AI is going to take over our jobs. Toss in references to machine learning, artificial neural networks (ANN), and multilayer ANN (aka deep learning), and it's difficult to know what to think about AI and how CISOs can assess whether the emerging technology is right for their organizations.
Gartner offers some suggestions on how to fight the FUD, as do Gartner security analysts Dr. Anton Chuvakin and Augusto Barros, who help demystifying AI in their blogs (not without a good note of sarcasm). In this piece, we will cover four practical questions a CISO should consider when investing AI-based products and solutions for cybersecurity.
Question 1: Do you have a risk-based, coherent, and long-term cybersecurity strategy?
Investing in AI without a crystal-clear, well-established, and mature cybersecurity program is like pouring money down the drain. You may fix one single problem but create two new ones or, even worse, overlook more dangerous and urgent issues.
A holistic inventory of your digital assets (i.e., software, hardware, data, users, and licenses) is the indispensable first step of any cybersecurity strategy. In the era of cloud containers, the proliferation of IoT, outsourcing, and decentralization, it is challenging to maintain an up-to-date and comprehensive inventory. However, most of your efforts and concomitant cybersecurity spending will likely be in vain if you omit this crucial step.
Every company should maintain a long-term, risk-based cybersecurity strategy with measurable objectives and consistent intermediary milestones; mitigating isolated risks or rooting out individual threats will not bring much long-term success. Cybersecurity teams should have a well-defined scope of tasks and responsibilities paired with the authority and resources required to attain the goals. This does not mean you should pencil implausibly picture-perfect goals, but rather agree with your board on its risk appetite and ensure incremental implementation of corporate cybersecurity strategy in accordance with it.
Question 2: Can a holistic AI benchmark prove ROI and other measurable benefits?
The primary rule of machine learning, a subset of AI, says to avoid using machine learning whenever possible. Joking aside, machine learning, capable of solving highly sophisticated problems that may have an indefinite number of inputs and thus outputs, is often prone to unreliability and unpredictability. It can also be quite expensive, with a return on investment years away – by which time the entire business model of a company could be obsolete.
For example, training datasets (discussed in the next question) may be costly and time-consuming to obtain, structure, and maintain. And, of course, the more untrivial and intricate the task, the more burdensome and costly it is to build, train, and maintain an AI model free from false positives and false negatives. In addition, businesses may face a vicious cycle when AI-based technology visibly reduces costs but requires disproportionally high investment for maintenance, which often exceeds the saved costs.
Finally, AI may be unsuitable for some tasks and processes where a decision requires a traceable explanation – for example, to prevent discrimination or to comply with law. Therefore, make sure you have a holistic estimate of whether implementation of AI will be economically practical both in short- and long-term scenarios.
Question 3. How much will it cost to maintain an up-to-date and effective AI product?
Cash is king on financial markets. In the business of AI, the royal regalia rightly belongs to the training datasets used to train a machine-learning model to perform different tasks.
The source, reliability, and sufficient volume of the training datasets are the primary issues for most AI products; after all, AI systems are only as good as the data we put into them. Often, a security product may require a considerable training period on-premises, assuming, among other things, that you have a riskless network segment that will serve as an example of the normal state of affairs for training purposes. A generic model, trained outside of your company, may simply be inadaptable for your processes and IT architecture without some complementary training in your network. Thus, make sure that training and the related time commitment are settled prior to product acquisition.
For most purposes in cybersecurity, AI products require regular updates to stay in line with emerging threats and attack vectors, or just with some novelties in your corporate network. Therefore, inquire how frequently updates are required, how long will they take to run, and who will manage the process. This may preclude a bitter surprise of supplementary maintenance fees.
Question 4. Who will bear the legal and privacy risks?
Machine learning may be a huge privacy peril. GDPR financial penalties are just a tip of the iceberg; groups and individuals whose data is unlawfully stored or processed may have a course of action against your company and claim damages. Additionally, many other applicable laws and regulations that may trigger penalties beyond GDPR's 4% revenue cap must be considered. Also keep in mind that most training datasets inevitably contain a considerable volume of PII, probably gathered without necessary consent or other valid basis. Worse, even if the PII is lawfully collected and processed, a data subject’s request to exercise one of the rights granted under the GDPR, such as right of access or right of erasure, can be unfeasible and the PII itself non-extractable.
Nearly 8,000 patents were filed in the United States between 2010 and 2018, 18% of which come from the cybersecurity industry. Hewlett Packard Enterprise warns about the legal and business risks related to unlicensed usage of patented AI technologies. So it might be a good idea to shift the legal risks of infringement to your vendors, for example, by adding an indemnification clause into your contract.
Few executives realize that their employers may be liable for multimillion-dollar damages for secondary infringement if a technology they use infringes an existing patent. The body of intellectual property law is pretty complicated, and many issues are still unsettled in some jurisdictions, bringing an additional layer of uncertainty about the possible outcomes of litigation. Therefore, make sure you talk to corporate counsel or a licensed attorney to get a legal advice on how to minimize your legal risks.
Last but not least, ascertain that your own data will not be transferred anywhere for "threat intelligence" or "training" purposes, whether legitimate or not.
Related Content:
Join Dark Reading LIVE for two cybersecurity summits at Interop 2019. Learn from the industry's most knowledgeable IT security experts. Check out the Interop agenda here.
About the Author(s)
You May Also Like
Beyond Spam Filters and Firewalls: Preventing Business Email Compromises in the Modern Enterprise
April 30, 2024Key Findings from the State of AppSec Report 2024
May 7, 2024Is AI Identifying Threats to Your Network?
May 14, 2024Where and Why Threat Intelligence Makes Sense for Your Enterprise Security Strategy
May 15, 2024Safeguarding Political Campaigns: Defending Against Mass Phishing Attacks
May 16, 2024
Black Hat USA - August 3-8 - Learn More
August 3, 2024Cybersecurity's Hottest New Technologies: What You Need To Know
March 21, 2024