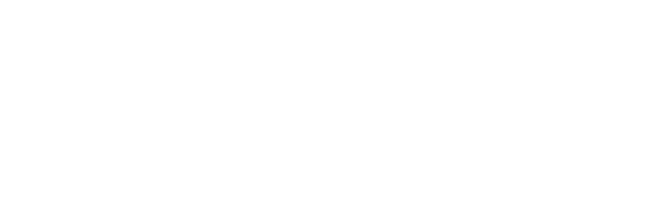
Cybersecurity In-Depth: Feature articles on security strategy, latest trends, and people to know.
The Hard Realities of Setting AI Risk Policy
It's time to get real about what it takes to set and enforce cybersecurity and resilience standards for AI risk management in the enterprise.
August 10, 2023
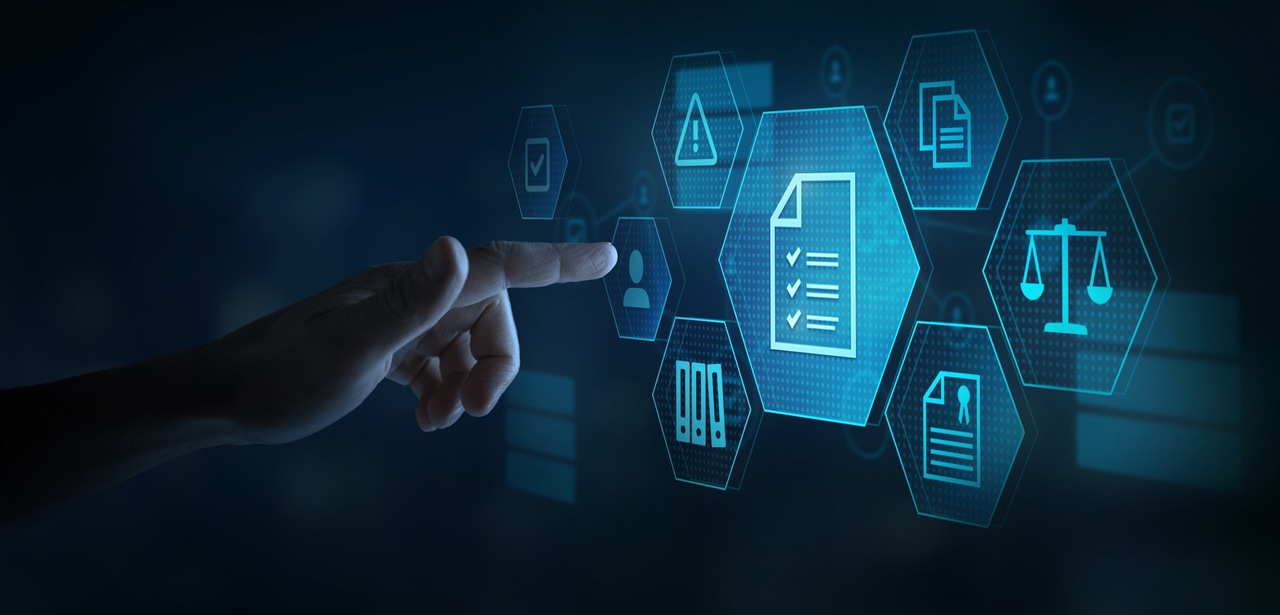
BLACK HAT USA – Las Vegas – Thursday, Aug. 10 – Here's some good news for artificial intelligence (AI) risk management: After years of warnings from cybersecurity, data science, and machine learning (ML) advocates, CISOs are finally paying attention. This is the year that cybersecurity professionals are waking up to the multilayered risks surrounding AI.
The hard part now is figuring out what comes next. What substantive steps do CISOs, executives, the board, and AI/ML developers need to take to set and enforce sane risk management policies?
That is the big question that a lot of attendees at Black Hat USA are asking, and it has threaded its way through a number of briefings and keynotes at this year's conference.
As the co-author of Not with a Bug, But with a Sticker: Attacks on Machine Learning Systems and What To Do About Them, Hyrum Anderson is a prolific AI security researcher and vocal advocate for raising awareness of AI risk and resilience issues. Anderson says the discussions at the podium and in the hallways at Black Hat are a continuation of what he saw earlier in the year at the RSA Conference (RSAC). Even if these problems aren't even close to being solved yet, he says he's just glad that the conversations are finally happening.
"A year ago, [my co-author Ram Shankar Siva Kumar] and I were shouting in the wind for CISOs to listen to us about AI risks. People would tell us this was science fiction, and they were more concerned about software supply chain and SolarWinds and whatever else," says Anderson, a distinguished ML engineer at Robust Intelligence. "Now things have changed drastically. At [RSAC] it felt like AI security had arrived. And there's a lot of excitement about the topic as we come into Black Hat."
The conference kicked off with keynotes and comments from Black Hat and DEF CON founder Jeff Moss and Azeria Labs founder Maria Markstedter, who explored the future of AI risk — which includes a raft of new technical, business, and policy challenges to navigate. The show features key briefings on research that has uncovered emerging new threats stemming from the use of AI systems, including flaws in generative AI that makes them prone to compromise and manipulation, AI-enhanced social engineering attacks, and how easily AI training data can be poisoned to impact the reliability of the ML models that depend on it. The latter, presented today by Will Pearce, AI red team lead for Nvidia, features research for which Anderson was a collaborator. He says the study shows that most training data is scoured from online sources that are easy to manipulate.
"The punchline is that for $60 we can control enough data to poison any model of consequence," he says.
For his part, Anderson is at Black Hat tackling some of the toughest technical challenges around discovering and quantifying risk from vulnerabilities in AI systems. This week he's spending his time at Black Hat Arsenal unveiling the newly open sourced AI Risk Database with collaborators from MITRE and Indiana University.
And what of those key questions around AI risk policy?
That's in the bailiwick of Anderson's book co-author Ram Shankar Siva Kumar, an affiliate at the Berkman Klein Center for Internet and Society at Harvard University, and Tech Policy Fellow at UC Berkeley. He took the podium yesterday with Jonathon Penney, associate professor for the Osgoode Hall Law School at York University, in their session "Risks of AI Risk Policy: Five Lessons." As public-private standards come out, like NIST AI RMF and the draft of the EU AI Act, enterprises are going to be facing tough choices about how and why they adhere to certain standards, Siva Kumar said. He emphasized that all of the standards were created by "very smart people" who are doing their best to pave the way with early guidelines for a very complex, rapidly changing AI landscape. The crux of his and Penney's talk revolved around five major realities of AI risk management policy adherence.
1. AI Systems Are Too Complex to Have Risk Managed by a Single, Unified Standard
"As I outlined the book with Hyrum, it's not as straightforward as, 'Hey, let's get a standard out, and let's have everybody snap onto it," Siva Kumar said, explaining that coming up with the AI equivalent of an Underwriter Labs (UL) safety rating used for electronics is not quite as simple to develop as it would be for, say, a toaster. "The first thing to understand is the technical reality of these AI systems is they're too complex to adhere to just one standard."
2. AI Standards Are Tough for Engineers to Parse
The second thing that CISOs need to keep in mind is that these policies are still very vague from a technical perspective, so implementing them will not be a matter of simply turning the text over to the engineering team and expecting them to follow a list. If you thought PCI compliance was tough in the early days, it's got nothing on this.
"Engineers find these AI standards pretty difficult to parse," Siva Kumar said.
3. Expect Dramatic Technical Trade-offs When Setting AI Risk Policies
One of the tough parts about AI policy development that all those smart people in the room are trying to figure out — and which is likely why there's still technical vagueness in guidelines — is that there are a ton of very dramatic technical trade-offs when it comes to instituting security and resilience measures in AI.
"There is no free lunch at the end of the day," said Siva Kumar, pointing out that existing adversarial ML research shows that even two basic factors, like robustness and bias, can sit on two ends of a teeter totter. Increase the robustness in a system and you could potentially impact the AI bias of the system — in fact, some research even shows that some measures to increase robustness may not be easily applied to all classes of data at the same rate of security.
"There's really a tension between the security and the other desired properties of AI," he said.
4. There Will Be Competing Interests in Achieving 'Good' AI Risk Management
Organizations are going to need solid leadership and a strong north star about their AI goals and the risks that matter most to them. Due to the technical trade-offs, there will be a lot of competing interests "warping how AI risk management solutions are being framed and sold and developed," Siva Kumar said.
5. Org Culture Is Key in Enforcing AI Risk Policy
One of the most important things that security leaders and the broader executive suite must keep in mind is that AI risk management isn't going to be a compliance exercise. None of these policies are a magic wand, and at the end of the day enterprise culture is going to have to become more collaborative and decisive in how they make AI risk decisions.
"You can have all these enforcement, these acts and regulations, but if the organization culture really does not change or adapt, and they continue to do what they do, all of this is going to be a great exercise in paperwork," Siva Kumar said.
Read more about:
Black Hat NewsAbout the Author(s)
You May Also Like
CISO Perspectives: How to make AI an Accelerator, Not a Blocker
August 20, 2024Securing Your Cloud Assets
August 27, 2024