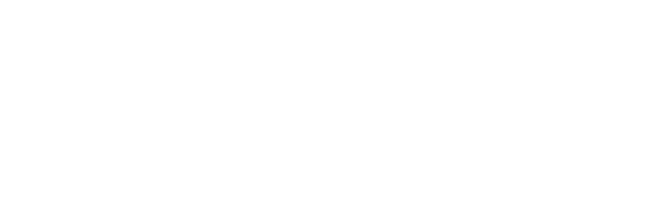
Cybersecurity In-Depth: Feature articles on security strategy, latest trends, and people to know.
Comparing Different AI Approaches to Email Security
There's a big difference between those that study 'known bads' and those that self-learn what is 'normal' and what isn't.
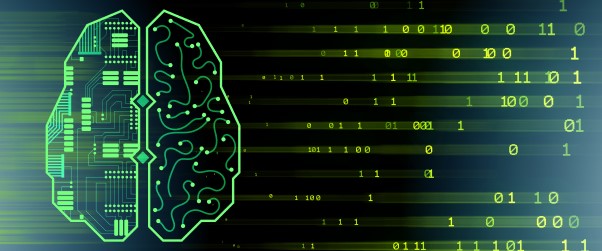
Innovations in artificial intelligence (AI) have fundamentally changed the email security landscape in recent years, but it can often be hard to determine what makes one system different than the next. In reality, under that umbrella term significant differences exist in approaches that may determine whether the technology provides genuine protection or simply a perceived notion of defense.
One backwards-looking approach involves feeding a machine thousands of emails that have already been deemed to be malicious, and training it to look for patterns in these emails in order to spot future attacks. The second approach uses an AI system to analyze the entirety of an organization's real-world data, enabling it to establish a notion of what is "normal" and then spot subtle deviations indicative of an attack.
In the below, we compare the relative merits of each approach, with special consideration to novel attacks that leverage the latest news headlines to bypass machine learning systems trained on data sets. Training a machine on previously identified "known bads" is only advantageous in certain, specific contexts that don't change over time: to recognize the intent behind an email, for example. However, an effective email security solution must also incorporate a self-learning approach that understands "normal" in the context of an organization in order to identify unusual and anomalous emails and catch even the novel attacks.
Signatures: a Backward-Looking Approach
Over the past few decades, cybersecurity technologies have looked to mitigate risk by preventing previously seen attacks from occurring again. In the early days, when the life span of a given strain of malware or the infrastructure of an attack was in the range of months and years, this method was satisfactory. But the approach inevitably results in playing catch-up with malicious actors: It always looks to the past to guide detection for the future. With decreasing lifetimes of attacks, where a domain could be used in a single email and never seen again, this historic-looking signature-based approach is now being widely replaced by more intelligent systems.
Training a Machine on 'Bad' Emails
The first AI approach we often see in the wild involves harnessing an extremely large data set with thousands or millions of emails. Once these emails have come through, an AI is trained to look for common patterns in malicious emails. The system then updates its models, rules set, and blacklists based on that data.
This method certainly represents an improvement to traditional rules and signatures, but it does not escape the fact that it is still reactive and unable to stop new attack infrastructure or new types of email attacks. It is simply automating that flawed, traditional approach – only, instead of having a human update the rules and signatures, a machine is updating them instead.
Relying on this approach alone has one basic but critical flaw: It does not enable you to stop new types of attacks it has never seen before. It accepts there has to be a "patient zero" – or first victim – in order to succeed.
The industry is beginning to acknowledge the challenges with this approach, and huge amounts of resources – both automated systems and security researchers – are being thrown into minimizing its limitations. This includes leveraging a technique called "data augmentation," which involves taking a malicious email that slipped through and generating many "training samples" using open source text augmentation libraries to create "similar" emails. This way, the machine learns not only the missed phish as "bad," but several others like it – enabling it to detect future attacks that use similar wording and fall into the same category.
But spending all this time and effort into trying to fix an unsolvable problem is like putting all your eggs in the wrong basket. Why try and fix a flawed system rather than change the game altogether? To spell out the limitations of this approach, let us look at a situation where the nature of the attack is entirely new.
The Rise of Fearware
When the global pandemic hit, and governments began enforcing travel bans and imposing stringent restrictions, there was undoubtedly a collective sense of fear and uncertainty. As explained in this blog, cybercriminals were quick to capitalize, taking advantage of people's desire for information to send out topical emails related to COVID-19 containing malware or credential-grabbing links.
These emails often spoofed the Centers for Disease Control and Prevention (CDC) and, later on, as the economic impact of the pandemic began to take hold, the Small Business Administration (SBA). As the global situation shifted, so did attackers' tactics. And in the process, more than 130,000 new domains related to COVID-19 were purchased.
Let's now consider how the above approach to email security might fare when faced with these new email attacks. The question becomes: How can you train a model to look out for emails containing "COVID-19" when the term hasn't even been invented?
And while COVID-19 is the most salient example of this, the same reasoning follows for every single novel and unexpected news cycle that attackers are leveraging in their phishing emails to evade tools using this approach – and attracting the recipient's attention as a bonus. Moreover, if an email attack is truly targeted to your organization, it might contain bespoke and tailored news referring to a very specific thing that supervised machine learning systems could never be trained on.
This isn't to say there's not a time and a place in email security for looking at past attacks to set yourself up for the future. It just isn't here.
Spotting Intention
Darktrace observes historical data for one specific, future-proof use. It analyzes emails' grammar and tone – which are not prone to change over time – in order to identify intent.
The AI asks questions like, "Does this look like an attempt at inducement? Is the sender trying to solicit some sensitive information? Is this extortion?" By training a system on an extremely large data set collected over a period of time, you can start to understand what, for instance, inducement looks like. This then enables you to easily spot future scenarios of inducement based on a common set of characteristics.
Training a system in this way works because, unlike news cycles and the topics of phishing emails, fundamental patterns in tone and language don't change over time. An attempt at solicitation is always an attempt at solicitation.
For this reason, this approach only plays one small part of a very large engine. It gives an addition indication about the nature of the threat but is not in itself used to determine anomalous emails.
Detecting the Unknown Unknowns
In addition to using the above approach to identify intention, Darktrace uses unsupervised machine learning, which starts with extracting and extrapolating thousands of data points from every email. Some of these are taken directly from the email itself, while others can only be ascertained by the above intention-type analysis. Additional insights are also gained from observing emails in the wider context of all available data across email, the network, and the cloud environment of the organization.
Only after having a now significantly larger and more comprehensive set of indicators, with a larger, more complete description of that email, can the data be fed into a topic-indifferent machine learning engine to start questioning the data in millions of ways in order to understand whether it belongs, given the wider context of the typical "pattern of life" for the organization. Monitoring all emails in conjunction allows the machine to establish things like:
Does this person usually receive zip files?
Does this supplier usually send links to Dropbox?
Has this sender ever logged in from China?
Do these recipients usually get the same emails together?
The technology identifies patterns across an entire organization and gains a continuously evolving sense of "self" as the organization grows and changes. It is this innate understanding of what is and isn't "normal" that allows AI to spot the truly "unknown unknowns" instead of just "new variations of known bads."
This type of analysis brings an additional advantage in that it is language- and topic-agnostic: Because it focuses on anomaly detection rather than finding specific patterns that indicate threat, it is effective regardless of whether an organization typically communicates in English, Spanish, Japanese, or any other language.
By layering both of these approaches, you can understand both the intention behind an email and whether the intention is for legitimate or malevolent purposes. And all of this is done without ever making an assumption or having the expectation you've seen this threat before.
Years in the Making
It's well-established now that the legacy approach to email security has failed – and this makes it easy to see why existing recommendation engines are being applied to the cybersecurity space. On first glance, these solutions may be appealing to a security team, but highly targeted, truly unique spear-phishing emails easily skirt by these systems. They can't be relied on to stop email threats on the first encounter because they have a dependency on known attacks with previously seen topics, domains, and payloads.
An effective, layered AI approach takes years of research and development. There is no single mathematical model to solve the problem of determining malicious emails from benign communication. A layered approach accepts that competing mathematical models each have their own strengths and weaknesses. It autonomously determines the relative weight these models should have and weighs them against one another to produce an overall "anomaly score" given as a percentage, indicating exactly how unusual a particular email is in comparison to the organization's wider email traffic flow.
It is time for email security to drop the assumption that you can look at threats of the past to predict tomorrow's attacks. An effective AI cyber security system can identify abnormalities with no reliance on historical attacks, enabling it to catch truly unique novel emails on the first encounter – before they land in the inbox.
This article was written by Dan Fein, director of email security products for the Americas at Darktrace. Based in New York, Dan joined Darktrace's technical team in 2015, helping customers quickly achieve a complete and granular understanding of Darktrace's world-leading Cyber AI Platform and products. Dan has a particular focus on Antigena Email, ensuring that it is effectively deployed in complex digital environments, and works closely with the development, marketing, sales, and technical teams. Dan holds a bachelor's degree in computer science from New York University.
About the Author(s)
You May Also Like
CISO Perspectives: How to make AI an Accelerator, Not a Blocker
August 20, 2024Securing Your Cloud Assets
August 27, 2024