Black Hat Europe 2016: How Artificial Intelligence Will Liberate Security
The security paradigm is shifting to one of 'security as a science' with AI as the primary agent for that revolutionary change.
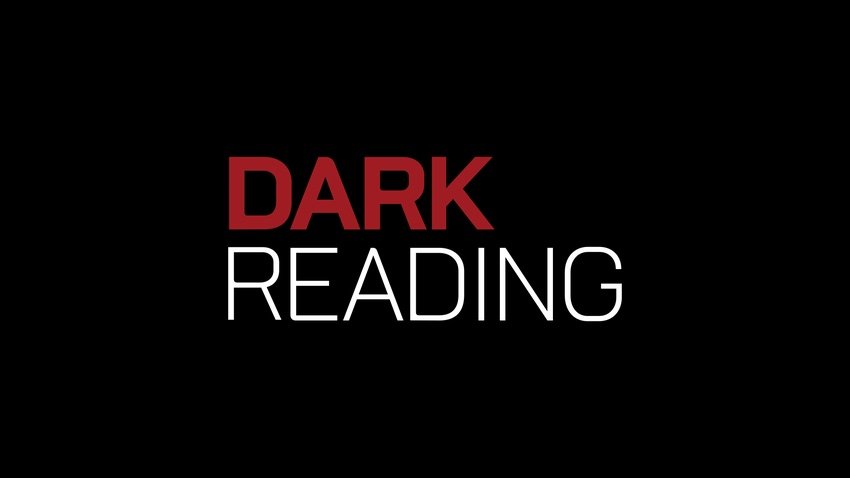
T. Kuhn's The Structure of Scientific Revolution outlined an episodic model in which periods of “normal science” were interrupted by periods of “revolutionary science.” It challenges us as a society to consider new paradigms, to change the rules of the game, our standards and our best practices.
The advent of applied Artificial Intelligence (AI), also known as machine learning, liberatingly delivers this new paradigm for malware prevention.
While legacy antivirus (AV) vendors had hoped that post-execution analysis solutions would give them an edge against the malware onslaught, it’s clear that relying on products that only detect malware after it has executed are no longer viable – but AI now provides us the opportunity to develop pre-execution malware prevention engines.
How it Works
The goal of pre-execution methodology is to analyze suspect code and determine if a file is good or bad based purely on the information contained in the file itself, and then repeat that at a sustainable massive scale.
Over the past few decades, patterns have emerged that dictate how specific types of files are constructed. There is variability in these patterns as well as anomalies, but as a whole, consistency has arisen as sample sizes increased.
Given the magnitude of the data involved, humans are simply incapable of leveraging it all to make a determination as to whether the file is malicious or not – yet legacy AV vendors still rely heavily on human decision-making in their processes.
Alternatively, machine learning focuses on prediction based on properties learned from earlier data to differentiate malicious files from safe or legitimate ones. The ability to do this across a huge number of samples is important because modern polymorphic malware creation is largely automated, enabling it to elude legacy AV solutions.
Applied AI uses complex algorithms that can predict if a program is malicious based on millions of feature sets. This approach to prevention has proven extremely effective at stopping malware before it gains a hold on a system, and without the need for initial victim(s) to become infected.
The Future of Malware Prevention
In order to keep up with modern attackers, security technologies need to evolve alongside them –without relying on human intervention. That’s where AI and machine learning have the advantage.
For years, industries such as healthcare, insurance, and high-frequency trading have applied the principals of AI and machine learning to analyze enormous quantities of business data and drive autonomous decision making.
Similarly, at the core of the AI-based security methodology is a massively scalable data-processing ‘brain’ capable of applying highly-tuned algorithmic models to enormous amounts of data in near real-time. An AI or machine learning approach to security will fundamentally change the way we understand and control risks posed by malicious code.
Much like Kuhn’s model predicted, the security paradigm is shifting from that of regular outmoded strategies to one of ‘security as a science,’ and AI is the primary agent for that revolutionary change.
Cylance – Protecting Everyone Under the Sun
About the Author(s)
You May Also Like
Key Findings from the State of AppSec Report 2024
May 7, 2024Is AI Identifying Threats to Your Network?
May 14, 2024Where and Why Threat Intelligence Makes Sense for Your Enterprise Security Strategy
May 15, 2024Safeguarding Political Campaigns: Defending Against Mass Phishing Attacks
May 16, 2024Why Effective Asset Management is Critical to Enterprise Cybersecurity
May 21, 2024
Black Hat USA - August 3-8 - Learn More
August 3, 2024Cybersecurity's Hottest New Technologies: What You Need To Know
March 21, 2024