Is Bias in AI Algorithms a Threat to Cloud Security?
Using AI for threat detection and response is essential — but it can't replace human intelligence, expertise, and intuition.
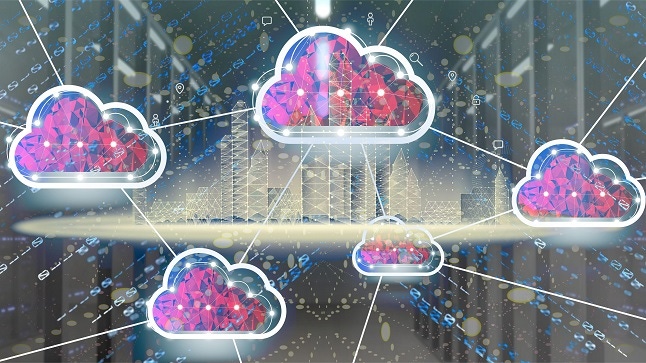
Artificial intelligence (AI) has been helping humans in IT security operations since the 2010s, analyzing massive amounts of data quickly to detect the signals of malicious behavior. With enterprise cloud environments producing terabytes of data to be analyzed, threat detection at the cloud scale depends on AI. But can that AI be trusted? Or will hidden bias lead to missed threats and data breaches?
Bias in Cloud Security AI Algorithms
Bias can create risks in AI systems used for cloud security. There are steps humans can take to mitigate this hidden threat, but first, it's helpful to understand what types of bias exist and where they come from.
Training data bias: Suppose the data used to train AI and machine learning (ML) algorithms is not diverse or representative of the entire threat landscape. In that case, the AI may overlook threats or identify benign behavior as malicious. For example, a model trained on data skewed toward threats from one geographical region might not identify threats originating from different regions.
Algorithmic bias: AI algorithms themselves can introduce their form of bias. For example, a system that uses pattern matching may raise false positives when a benign activity matches a pattern or fail to detect subtle variations in known threats. An algorithm can also be tuned inadvertently to favor false positives, leading to alert fatigue, or to favor false negatives, allowing threats to get through.
Cognitive bias: People are influenced by personal experience and preferences when processing information and making judgments. It's how our minds work. One cognitive bias is to favor information that supports our current beliefs. When people create, train, and fine-tune AI models, they can transfer this cognitive bias to AI, leading the model to overlook novel or unknown threats such as zero-day exploits.
Threats to Cloud Security from AI Bias
We refer to AI bias as a hidden threat to cloud security because we often don't know that bias is present unless we specifically look for it — or until it is too late and a data breach has happened. Here are some of the things that can go wrong if we fail to address bias:
Inaccurate threat detection and missed threats: When training data is not comprehensive, diverse, and current, the AI system can over-prioritize some threats while under-detecting or missing others.
Alert fatigue: Overproduction of false positives can overwhelm the security team, potentially causing them to overlook genuine threats that get lost in the volume of alerts.
Vulnerability to new threats: AI systems are inherently biased because they can only see what they've been trained to see. Systems that are not kept current via continuous updating and equipped with the ability to learn continuously will not protect cloud environments from newly emerging threats.
Erosion of trust: Repeated inaccuracies in threat detection and response due to AI bias can undermine stakeholder and security operations center (SOC) team trust in the AI systems, affecting cloud security posture and reputation long term.
Legal and regulatory risk: Depending on the nature of the bias, the AI system might violate legal or regulatory requirements around privacy, fairness, or discrimination, resulting in fines and reputational damage.
Mitigating Bias and Strengthening Cloud Security
While humans are the source of bias in AI security tools, human expertise is essential to building AI that can be trusted for securing the cloud. Here are steps that security leaders, SOC teams, and data scientists can take to mitigate bias, foster trust, and realize the enhanced threat detection and accelerated response that AI offers.
Educate security teams and staff about diversity: AI models learn from the classifications and decisions analysts make in assessing threats. Understanding our biases and how they influence our decisions can help analysts avoid biased classifications. Security leaders can also ensure that SOC teams represent a diversity of experiences to prevent blind spots that result from bias.
Address the quality and integrity of training data: Employ robust data collection and preprocessing practices to ensure that training data is free of bias, represents real-world cloud scenarios, and covers a comprehensive range of cyber threats and malicious behaviors.
Account for the peculiarities of cloud infrastructure: Training data and algorithms must accommodate public cloud-specific vulnerabilities, including misconfigurations, multi-tenancy risks, permissions, API activity, network activity, and typical and anomalous behavior of humans and nonhumans.
Keep humans "in the middle" while leveraging AI to fight bias: Dedicate a human team to monitor and evaluate the work of analysts and AI algorithms for potential bias to make sure the systems are unbiased and fair. At the same time, you can employ specialized AI models to identify bias in training data and algorithms.
Invest in continuous monitoring and updating: Cyber threats and threat actors evolve rapidly. AI systems must learn continuously, and models should be regularly updated to detect new and emerging threats.
Employ multiple layers of AI: You can minimize the impact of bias by spreading the risk across multiple AI systems.
Strive for explainability and transparency: The more complex your AI algorithms are, the more difficult it is to understand how they make decisions or predictions. Adopt explainable AI techniques to provide visibility into the reasoning behind AI outcomes.
Stay on top of emerging techniques in mitigating AI bias: As we progress in the AI domain, we're witnessing a surge in techniques to spot, quantify, and address bias. Innovative methods like adversarial de-biasing and counterfactual fairness are gaining momentum. Staying abreast of these latest techniques is paramount in developing fair and efficient AI systems for cloud security.
Ask your managed cloud security services provider about bias: Building, training, and maintaining AI systems for threat detection and response is hard, expensive, and time-consuming. Many enterprises are turning to service providers to augment their SOC operations. Use these criteria to help evaluate how well a service provider addresses bias in AI.
The Takeaway
Given the scale and complexity of enterprise cloud environments, using AI for threat detection and response is essential, whether in-house or outside services. However, you can never replace human intelligence, expertise, and intuition with AI. To avoid AI bias and protect your cloud environments, equip skilled cybersecurity professionals with powerful, scalable AI tools governed by strong policies and human oversight.
About the Author(s)
You May Also Like
Is AI Identifying Threats to Your Network?
May 14, 2024Where and Why Threat Intelligence Makes Sense for Your Enterprise Security Strategy
May 15, 2024Safeguarding Political Campaigns: Defending Against Mass Phishing Attacks
May 16, 2024Why Effective Asset Management is Critical to Enterprise Cybersecurity
May 21, 2024Finding Your Way on the Path to Zero Trust
May 22, 2024
Black Hat USA - August 3-8 - Learn More
August 3, 2024Cybersecurity's Hottest New Technologies: What You Need To Know
March 21, 2024