A New Era Is Dawning in Cybersecurity, but Only the Best Algorithms Will Win
Open source AI is lowering the barrier of entry for cybercriminals. Security teams must consider the right way to apply defensive AI to counter this threat.
January 17, 2023
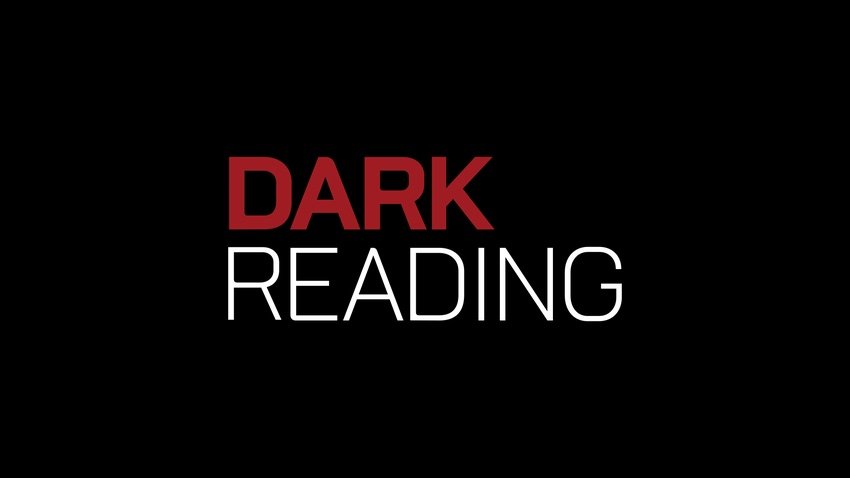
In the wake of increasing concern about threat actors using open source AI tools like ChatGPT to launch sophisticated cyberattacks at scale, it's time for us to reconsider how AI is being leveraged on the defensive side to fend off these threats.
Ten years ago, cybersecurity was a different ball game. Threat detection tools typically relied on "fingerprinting" and looking for exact matches with previously encountered attacks. This "rearview mirror" approach worked for a long time, when attacks were lower in volume and generally more predictable.
Over the last decade, attacks have become more sophisticated and ever-changing on the offensive side. On the defensive side, this challenge is coupled with complex supply chains, hybrid working patterns, multicloud environments, and IoT proliferation.
The industry recognized that basic fingerprinting could not keep up with the speed of these developments, and the need to be everywhere, at all times, pushed the adoption of AI technology to deal with the scale and complexity of securing the modern business. The AI defense market has since become crowded with vendors promising data analytics, looking for "fuzzy matches": near matches to previously encountered threats, and ultimately, using machine learning to catch similar attacks.
While an improvement to basic signatures, applying AI in this way does not escape the fact that it is still reactive. It may be able to detect attacks that are highly similar to previous incidents, but remains unable to stop new attack infrastructure and techniques that the system has never seen before.
Whatever label you give it, this system is still being fed that same historical attack data. It accepts that there must be a "patient zero" — or first victim — in order to succeed.
The "pretraining" of an AI on observed data is also known as supervised machine learning (ML). And indeed, there are smart applications of this method in cybersecurity. In threat investigation, for example, supervised ML has been used to learn and mimic how a human analyst conducts investigations — asking questions, forming and revising hypotheses, and reaching conclusions — and can now autonomously carry out these investigations at speed and scale.
But what about finding the initial breadcrumbs of an attack? What about finding the first sign that something is off?
The problem with using supervised ML in this area is that it's only as good as its historical training set — but not with things it's never seen before. So it needs to be constantly updated, and that update needs to be pushed to every customer. This approach also requires the customer's data to be sent off to a centralized data lake in the cloud, for it to be processed and analyzed. By the time an organization knows about a threat, often it's too late.
As a result, organizations suffer from a lack of tailored protection, large numbers of false positives, and missed detections because this approach is missing one crucial thing: the context of the unique organization it is tasked with protecting.
But there is hope for defenders in the war of algorithms. Thousands of organizations today use a different application of AI in cyber defense, taking a fundamentally different approach to defend against the entire attack spectrum — including indiscriminate and known attacks but also targeted and unknown attacks.
Rather than training a machine on what an attack looks like, unsupervised machine learning involves the AI learning the organization. In this scenario, the AI learns its surroundings, inside and out, down to the smallest digital details, understanding "normal" for the unique digital environment it's deployed in to identify what's not normal.
This is AI that understands "you" in order to know your enemy. Once considered radical, today it defends over 8,000 organizations worldwide by detecting, responding, and even preventing the most sophisticated cyberattacks.
Take the widespread Hafnium attacks exploiting Microsoft Exchange Servers last year. These were a series of new, unattributed campaigns that were identified and disrupted by Darktrace's unsupervised ML in real time across many of its customer environments without any prior threat intelligence associated with these attacks. In contrast, other organizations were left unprepared and vulnerable to the threat until Microsoft disclosed the attacks a few months later.
This is where unsupervised ML works best — autonomously detecting, investigating, and responding to advanced and never-before-seen threats based on a bespoke understanding of the organization being targeted.
At Darktrace, we have tested this AI technology against offensive AI prototypes at our AI research center in Cambridge, UK. Similar to ChatGPT, these prototypes can craft hyperrealistic and contextualized phishing emails and even select a fitting sender to spoof and fire the emails away.
Our conclusions are clear: As we start to see attackers weaponizing AI for nefarious purposes, we can be certain that security teams will need AI to fight AI.
Unsupervised ML will be critical because it learns on the fly, building a complex, evolving understanding of every user and device across the organization. With this bird's-eye view of the digital business, unsupervised AI that understands "you" will spot offensive AI as soon as it starts to manipulate data and will make intelligent microdecisions to block that activity. Offensive AI may well be leveraged for its speed, but this is something that defensive AI will also bring to the arms race.
When it comes to the war of algorithms, taking the right approach to ML could be the difference between a robust security posture and disaster.
About the Author
.jpg/?width=700&auto=webp&quality=80&disable=upscale)
Tony Jarvis is Director of Enterprise Security, Asia-Pacific and Japan, at Darktrace. Tony is a seasoned cybersecurity strategist who has advised Fortune 500 companies around the world on best practice for managing cyber-risk. He has counseled governments, major banks, and multinational companies, and his comments on cybersecurity and the rising threat to critical national infrastructure have been reported in local and international media, including CNBC, Channel News Asia, and The Straits Times. Tony holds a BA in Information Systems from the University of Melbourne.
You May Also Like
Key Findings from the State of AppSec Report 2024
May 7, 2024Is AI Identifying Threats to Your Network?
May 14, 2024Where and Why Threat Intelligence Makes Sense for Your Enterprise Security Strategy
May 15, 2024Safeguarding Political Campaigns: Defending Against Mass Phishing Attacks
May 16, 2024Why Effective Asset Management is Critical to Enterprise Cybersecurity
May 21, 2024
Black Hat USA - August 3-8 - Learn More
August 3, 2024Cybersecurity's Hottest New Technologies: What You Need To Know
March 21, 2024