Machine Learning Is the Next Great Security Weapon
Enlisting machines will help level the playing field in the battle for enterprise cybersecurity.
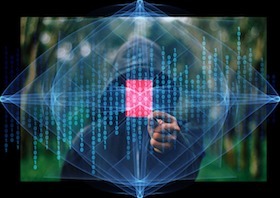
In cybersecurity, there are three certainties: hackers will always get smarter, their frequency will accelerate and eventually they will get in.
Today, most mature enterprises and seasoned security professionals operate from a reactive posture, seeking and responding to threats as best they can. But a Security Operations Center (SOC) is only responsive to what they can readily identify, and there is simply too great of an imbalance between the amount of data to be analyzed and IT staff to monitor everything.
Enter machine learning (ML). ML is bringing security operations closer to an even playing field with cyber criminals. Within five years, it will be the driving force for security detection and defense, a tool that never ceases monitoring for anomalies that can be signs of malicious activity from inside or outside the organization.
ML is emerging as the preferred toolset for enabling operational decisions to optimize IT, security and business operations. In security, it can equip IT to better detect incidents, reduce resolution times, automate responses and protect an organization’s most valuable information.
Adapt and adopt
Security threats are becoming more powerful. Ransomware can be fearsome, with the potential to paralyze the operations of large global enterprises or smaller organizations with fewer defenses. Improved techniques make ransomware a more legitimate day-to-day threat that makes victimized organizations choose between having their operations frozen or erased, or paying a sum to be released.
In addition, there are more large-scale attacks that employ botnets, using innocuous tech like routers and Internet of things connected devices, to overwhelm an online presence with a flood of Internet traffic. It's likely large, purposeful DDoS attacks against the Internet will increase and have the potential to be debilitating for certain sectors that must be online 24/7 such as healthcare, government and utilities.
It’s also notable that hackers now seek access to extract data not only to monetize on it, but to weaponize it. Leaks that damage reputation or reveal proprietary information are commonly used publicity tactics that can disrupt an organization beyond taking a financial hit. Enter ML.
Machine learning wins
This evolution of the market makes analytics-driven security strategies built on data an absolute imperative. Businesses are looking for new strategies to maximize the value of their massive influx of data, which introduces automation as a fundamental driver of how they operate. A large percentage of the data that businesses deal with is now generated by machines -- servers, sensors, firewalls and other devices. Some of the most advanced ML algorithms available today are built to make better use of that data.
ML allows organizations to be able to better analyze attacks happening right now, rather than looking for past trends. ML is used not only for identifying patterns that can indicate an attack, but for tasks such as tracking multiple parameters across different areas of the business in real time. Whereas the challenge for security to date could be described as searching for the needle in the haystack, today’s SOC is tasked with finding the oddly shaped needle in a gigantic pile of needles.
Traditional analytics systems may seem to perform well, but weren’t built to analyze and learn from machine data. That falls to human workers, and in most organizations, there are simply not enough personnel to handle this work. ML can automate searching for anomalies in behavior or activity, and alert security teams to the highest priority concerns. This allows organizations to automate detection and response to both known and unknown threats.
One stubbornly difficult challenge that adoption of ML can take on is the insider threat. For example, a recent survey by Dell revealed a shockingly high number of employees (72%) said they would be willing to share confidential information. Malicious insider threats are so persistent because they vary by organization and are too difficult to govern by static correlation searches. ML can make identification of insider threats more easily discoverable, and amplify and augment security analysts’ ability to work on such high-value problems.
ML's gravitational pull
It seems likely that SOCs of the future will have ML at the core, incorporate it for threat detection, risk analysis, prevention and incident response. ML is already fused with critical security technologies like security information and event management (SIEM) and user-behavior analytics (UBA). This convergence will help create security that’s more dynamic and agile, and focused on long-term, analytics-driven threat-hunting with machine learning. Security analysts will still be necessary to apply human intelligence to machine data, however the benefits provided by machine learning and automation will enable them to build a stronger, proactive security strategy.
Companies seeking machine learning for security should carefully screen vendors to ensure they're getting what they pay for. The market is currently rife with confusion -- vendors can be ignorant or disingenuous when it comes to the use of the term machine learning. For instance, what is marketed as ML may just be a basic detection tool with signatures.
There are also significant differences between advanced and basic ML offerings. Sophisticated ML should enable focused investigation, intelligent alerting and predictive actions.
To successfully implement machine learning for security, a business must begin with an analytics platform that is well suited to delivering business insights from machine data.
As more companies utilize ML that is highly customized to their organization, security professionals will evolve with it.
— Haiyan Song is Senior Vice President of Security Markets for Splunk.
Read more about:
Security NowAbout the Author(s)
You May Also Like
CISO Perspectives: How to make AI an Accelerator, Not a Blocker
August 20, 2024Securing Your Cloud Assets
August 27, 2024