Mastering Security Analytics
Fast data analysis can stymie attacks and strengthen enterprise security. Does your team have the data smarts?
October 14, 2014
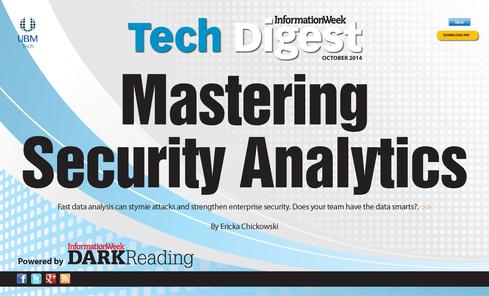
Download the latest issue of Dark Reading Tech Digest (registration required).
Today's enterprise security tools have developed an ability to detect a plethora of anomalies and "events" that indicate an attack is underway. For most companies, the problem is interpreting all of that security data to identify sophisticated threats and eliminate them before a serious data loss occurs.
"We're sort of living in this alert-driven culture, but no one has the resources to respond to every alert," says Dmitri Alperovitch, co-founder and CTO of CrowdStrike, a security intelligence and analytics firm. "There are a lot of false positives, so not every alert is going to be prioritized."
Innovations within security software, appliances, and services have automated many detection and blocking tasks, resulting in improved protection from next-generation firewalls and intrusion-prevention systems. But no matter how advanced a tool is, it will never block 100% of attacks.
That's why, even with so much sophisticated technology available today, brainpower remains the most effective tool in fighting advanced attacks. Smart analysts can connect the dots among different security alerts and logs, letting analysts hunt down and shut down the sneakiest of exploits. But as security data proliferates, these analysts are being snowed under.
Even the most highly skilled analysts can only sift through so much data per day before they become ineffective. What's more, there are only so many analysts out there -- and they don't come cheap.
For most companies, then, it's not just a matter of hiring more analysts. "It's all about how do you maximize the efficiency of your human analysts -- how you present them with the information that's most relevant to them and most actionable," Alperovitch says.
To do that, IT organizations must rethink the factors that drive their security intelligence and analysis. They need to find ways to digest data more efficiently and automate some of the easier correlations among data sets so that analysts have more time to focus on the complex ones.
There are a number of ways to improve data analysis, and much of it revolves around providing data in better context, automating data flows and mathematical analyses, and improving the way data is presented to humans when it's decision-making time.
The trouble with SIEM
Anyone who has been in IT security for a little while might stop at this point and ask, "Wait, isn't data analysis what security information and event management (SIEM) systems are for?"
When SIEM technology kicked off over a decade ago, the promise was that these platforms would become the catch-all system for storing and correlating security data across the enterprise to help analysts stop attacks in their tracks. But that was a time when the corporate attack surface was fairly limited, and the volume of attacks was still manageable. Many of these SIEM systems had a pedigree in log management, and their underlying architecture was built in a time long before the nonrelational database revolutionized big data analysis. As a result, SIEM has a number of weaknesses that keep it from being an analytical superstar.
First, many SIEM platforms still can't pull in all of the necessary feeds to track attacks across the typical attack life cycle, or kill chain, which often spans endpoints, network resources, databases, and so on. Even when they can ingest data from, say, endpoint security systems, they are often unable to normalize it (meaning get the data sets into roughly the same format) and pair it with related network security data that could help analysts correlate separate events into a single attack.
"The challenge is you have endpoint systems that don't talk to log data and don't talk to network data," says Craig Carpenter of AccessData, a forensics and incident response vendor. "It may all be sitting in the SIEM, but it's not being correlated. It's not being translated into a singular language that the analyst can actually look at."
In most cases, Carpenter adds, you'll have two different teams looking at the data: the network team and the endpoint team.
"And the two alerts don't match to each other, so they look like completely different events to the analysts," he says.
As the number of security data feeds increases with more specialized services and products -- be they phishing and malware detection or external threat intelligence data -- it only gets harder to map out a single attack across all of the different infrastructure touch points. It's a case of too many alerts with little to no context.
"There's no prioritization," explains Alperovitch. "So it's easy to say with hindsight that they should have connected the dots because there was one alert, but if there's 5 million dots for you to connect, then it's really, really hard for any organization to make sense of it all."
For example, prior to its breach, the retailer Target did get an alert from its security tool, but it was lost in the noise of many other alerts coming in at a rate of hundreds a day.
To read the rest of this story, download the latest issue of
Dark Reading Tech Digest (registration required).
About the Author(s)
You May Also Like
Is AI Identifying Threats to Your Network?
May 14, 2024Where and Why Threat Intelligence Makes Sense for Your Enterprise Security Strategy
May 15, 2024Safeguarding Political Campaigns: Defending Against Mass Phishing Attacks
May 16, 2024Why Effective Asset Management is Critical to Enterprise Cybersecurity
May 21, 2024Finding Your Way on the Path to Zero Trust
May 22, 2024
Black Hat USA - August 3-8 - Learn More
August 3, 2024Cybersecurity's Hottest New Technologies: What You Need To Know
March 21, 2024