New 'Mac-A-Mal' Tool Automates Mac Malware Hunting & Analysis
Researchers at Black Hat Asia will demonstrate a new framework they created for catching and studying Apple MacOS malware.
March 14, 2018
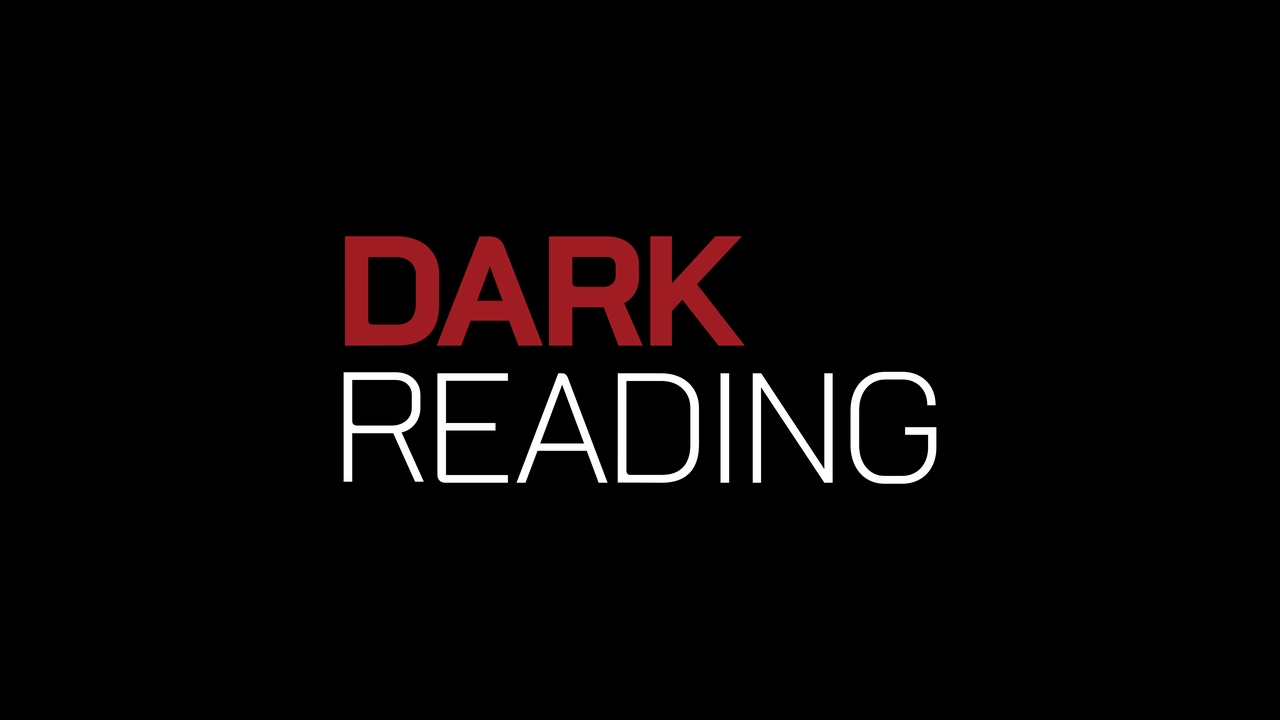
Malware targeting Windows machines still dominates the threat landscape, but hackers gradually have been expanding their target range to increasingly popular Apple MacOS platforms. A team of researchers now has created an automated MacOS malware analyzer that streamlines and simplifies the process of detecting and studying the growing ecosystem of malicious code targeting Macs.
MacOS research tools typically have relied on manual analysis of malware, notes Pham Duy Phuc, a malware analyst with Netherlands-based Sfylabs BV. Phuc says he first began developing the so-called Mac-A-Mal tool while pursuing his Master's Degree at the University of Trento in Italy.
"There are tools for malware reverse-engineering, debugging, and malware analysis on Mac," including commercial tools like Hopper and IDA, and open-source tools like Radare2, MachO View, lldb, Otool, and Dtrace, Phuc noted in an email interview. But these tools mostly require manual analysis, which means the researcher also must have some know-how in order to use them.
"Each tool only solves one piece of the puzzle and it depends on experience of the researcher. Using these tools manually takes too much time and effort, and will never combat malicious software," said Phuc. "For a demand of thousands [of] malware per day, an automated framework with combination of useful tools would make malware analyst daily job easier."
Phuc and Fabio Massacci, his former professor at the University of Trento, will demonstrate Mac-A-Mal at Black Hat Asia in Singapore next week. The two also plan to soon release the tool as open-source.
[See researchers demonstrate Mac-A-Mal live at Black Hat Asia in Singapore next week, March 22-23: conference and registration information.]
Mac-A-Mal uses a combination of static- and dynamic code analysis to detect MacOS malware, as well as to cheat anti-analysis methods that some malware authors use to evade detection and investigation. It gathers malware binary behavior patterns, such as network traffic, evasion methods, and file operation. The tool uses kernel-level system calls, which allows it to operate undetected. "It takes actual behavioral data of malware samples, executions, inside a sandbox," he said.
Half of Mac Malware = Backdoors
The researchers used the tool to parse some 2,000 Mac samples on VirusTotal, which led to the discovery of a previously unknown adware campaign that uses legitimate Apple developer certificates, keyloggers, and Trojans. They believe the adware operation is the handiwork of the APT32 aka OceanLotus group believed to be out of Vietnam, and it's targeting Chinese and Vietnamese organizations.
"By studying the first generation of Mac OceanLotus samples through our framework, we found some similar behavioral signatures amongst the family. In March 2017, we found a second generation of Mac APT32 which [has a] zero-detection rate over more than 50 antivirus vendors ... hunting those behaviors on VirusTotal," he said. That new variant is more advanced, he said.
Phuc says the team also discovered hundreds of other Mac malware samples that with manual tools would be difficult to identify, and nearly half of all Mac malware collected in 2017 on VirusTotal were backdoor Trojans. The majority of malware samples were adware, mostly OSX/Pirrit and OSX/MacKeeper. "We observed a total of 86 different Mac malware families until 2017, and 49% of them belongs to backdoor/Trojan" categories, he said.
Mac-A-Mal basically works like this: it finds MacOS malware and places the samples in a sandbox where it performs static analysis on multiple samples at the same time. "The sandbox is armored with network sniffer, system calls and behavior logging, as well as anti-evasion from kernel-mode to send back a report to analysis machine," Phuc explained.
Kernel-level monitoring has its advantages, according to Phuc. Namely it's a more complete view from the lower level of the operating system, while at the same time keeping Mac-A-Mal under cover from anti-analysis detection. Next up for Mac-A-Mal is machine learning capabilities: "We would like to later apply more robust and advanced techniques for better features extraction from the analysis, and machine learning for a larger scale of Mac samples," Phuc said.
Black Hat Asia returns to Singapore with hands-on technical Trainings, cutting-edge Briefings, Arsenal open-source tool demonstrations, top-tier solutions and service providers in the Business Hall. Click for information on the conference and to register.
Related Content:
Read more about:
Black Hat NewsAbout the Author
You May Also Like